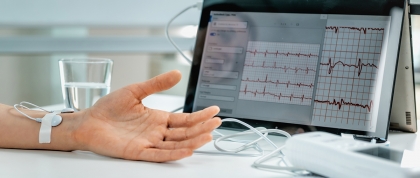
Cardiovascular disease (CVD) is the number one cause of death globally. It has been decreasing since the 1960’s in the Global North due to significant advances in both prevention and treatment, but these declining trends have stalled, or in some instances increased, among some racial/ethnic, low income and other vulnerable groups.
Today there are steep disparities in CVD outcomes and mortality in the U.S. and globally, and evidence shows that a substantial portion is attributable to social determinants of health such as income, social isolation, pollution, the walkability of a community and the availability of resources like grocery stores.
Machine learning—a statistical method used to detect patterns in data—is being rapidly developed in CVD research and statistical methods are already central in assessing disease risk and U.S. prevention guidelines. However, for some groups these methods fare poorly.
To address the need to build more comprehensive CVD risk prediction models, we performed a systematic review of research on machine learning and CVD risk that includes social determinants of health, and found that including them improved the ability to predict cardiovascular outcomes like rehospitalization, heart failure, and stroke.
However, we also found a lack of geographic diversity in the studies, as the majority used data from the U.S., Europe and China, neglecting parts of the world experiencing significant increases in cardiovascular disease. This limited knowledge generation will result in an inadequate understanding of how social determinants interact in different settings, and limit the applicability of CVD risk prediction models.
Including social determinants in CVD risk prediction models could help health professionals identify patients in need of referral to community resources like housing services, and will broadly focus attention to where in the risk structure we should promote intervention via local, state, and national policies.
Overall, changes in CVD burden and the landscape of technologies used in healthcare show how the public health community should be involved with technology development from the outset, to ensure they will be designed in a way that can work for all people.
Rumi Chunara, PhD
Associate Professor of Biostatistics
Stephanie Cook, DrPH
Assistant Professor of Biostatistics and Assistant Professor of Social and Behavioral Sciences