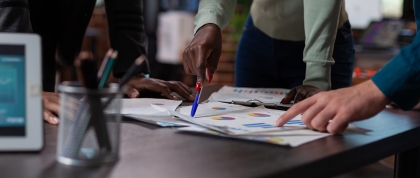
Neurological complications, and specifically toxic-metabolic encephalopathy (TME), are common among hospitalized COVID-19 patients, and cognitive symptoms persist in many. One hypothesis is that reduced oxygen and increased inflammation may lead to brain injury. To test this, my colleagues at the NYU Grossman School of Medicine and I studied levels of nine markers of neurodegeneration (brain damage) in hospitalized COVID-19 patients.
Five of these markers are specific for Alzheimer’s disease pathology; others are associated with blood-brain barrier disruption and with brain injury. We retrospectively analyzed COVID-19 patients who had serum collected and banked as part of a prospective acute COVID study conducted at NYU hospitals. These patients were hospitalized for COVID-19 during the period March 10, 2020 through May 20, 2020 and had no history of cognitive impairment or dementia.
In one set of analyses, we compared the markers measured at hospital admission between those with new neurological events (TME) in the hospital and those without such events in the hospital. We found that neurodegenerative biomarker levels were higher for patients who experienced TME in the hospital than for those who did not. We also compared those who died in the hospital versus those who were discharged from the hospital and found similar results. These results were published recently in Alzheimer’s and Dementia (Scitech Daily).
These results are interesting as they provide evidence of the association between neurodegenerative biomarkers and neurological complications of COVID-19. However, careful consideration of the results raises the question of the meaning of TME occurrence in the hospital. TME might have occurred while the patient was in the hospital because its onset was rapid. Alternatively, it might have occurred in the hospital because the patient was in the hospital for a long time due to the severity of their COVID-19, but not necessarily due to the severity of the TME. And surely there are other explanations, as well. The bottom line is that it’s not clear what it means to observe TME in a patient while in the hospital, and it might very well have several meanings.
Another way of stating this observation is that the comparisons between groups defined by TME in the hospital are intertwined with time spent in the hospital before death or discharge to a nursing home or to home. An alternative analysis is the comparison of the markers between patients who ever experience TME due to COVID-19 versus those who never experience TME due to COVID-19. And likewise, the comparison of the markers between patients who die due to COVID-19 versus those who do not die due to COVID-19. You might wonder how this would be possible given that all we observe is what occurs in the hospital.
In fact, it is possible given the variation in times to TME and times to in-hospital death among the patients. This allows us to consider TME occurrence and death that occurs after hospital discharge. In doing so, it is important to build into any model the possibility that patients might never experience TME due to COVID-19 and might never die from COVID-19.
Based on this so-called “cure model,” I was able to construct estimates for the distributions of the biomarkers for the groups of interest. It turns out that they can be quite different from the in-hospital TME distribution and from the in-hospital death distribution. For example, the median values of one Alzheimer’s disease marker were 14.4 and 12.7 for those who experienced TME in the hospital versus those who did not, while they were estimated to be 28.1 and 3.3 for those who experienced TME ever versus those who did not.
These analyses illustrate the importance of considering the constraints of the data in the interpretations. They also exemplify the possibility of exploiting features of the data, in conjunction with models that make minimal assumptions, to overcome the constraints and obtain improved interpretability.
Rebecca Betensky, PhD
Chair and Professor of Biostatistics