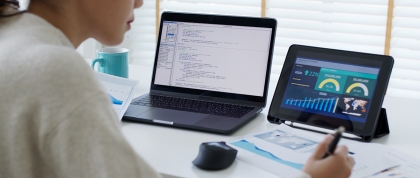
Last year I met Mina, a young female health data scientist who was working on cervical cancer burden estimates in Karachi, Pakistan — a challenging task due to the need to harmonize disparate datasets in lieu of public health registries. As she shared with me the difficulties that many Pakistani women face, Mina opened my eyes to how diseases — even genetic diseases — can be shaped by social structures. She showed me that remaining undiagnosed, unaware and unable to take preventative measures or treatment are all strongly influenced by forces including poverty, racism, stigma and patriarchy.
The fundamental need for data to measure and evaluate the efficacy of interventions is clear. At the same time we must remain vigilant, given the potential to exacerbate health disparities through the use of data. Biases in data and analysis are already part of daily life, from facial recognition that works less accurately for darker skinned women, to algorithms that provide more care for white patients than Black patients at the same level of sickness. At NYU’s Center for Health Data Science, we’re asking how we can prepare the next generation of public health professionals and researchers to best leverage data and underwrite risks.
In a new publication in Harvard Data Science Review, my co-authors and I examined public health data science courses and programs around the world. While many degree programs focus on machine learning or artificial intelligence, most public health schools offer zero or only one course in either data communication or the ethics of data use. Our detailed review highlighted an educational gap in the social and political dimensions of using data. These topics are integral not only to public health but also to disciplines such as socio-behavioral sciences, urban planning, engineering and anthropology.
Our previous work reinforced that a lack of diverse perspectives leads to poor outcomes, and that it’s critical to mitigate inequities. As Paul Farmer said, and the recent Covid-19 pandemic and massive, climate-driven floods around the globe have shown, “We live in one world.” Data science needs multiple perspectives to best address health challenges. So it was sad for me to see that Mina, discouraged, soon left her position in Karachi. While data science training is becoming more commonplace and available, the right institutional support is critical for individuals to grow professionally and achieve their goals.
One such example is the NYU-Moi Data Science for Social Determinants Training Program, which is simultaneously advancing data science capacity at Moi University in Western Kenya and creating pathways for collaborative research at NYU. With this cross-fertilization, and a paradigm shift of what concepts we capture in data, we hope to improve outcomes. Certainly, if structural factors are not accounted for in data, we cannot assess their impact.
As a resource for a holistic approach to data, with a diversity of people and forms of knowledge, the Center for Health Data Science represents NYU’s commitment to transdisciplinary work and transformative ideas. But we cannot achieve this vision alone. We invite you to join us so that collectively our ideas and spirit of inquiry will animate new horizons in public health data science.
Rumi Chunara, PhD
Associate Professor of Biostatistics; Associate Professor of Computer Science and Engineering, Tandon; Director of Center for Health Data Science