Hai Shu
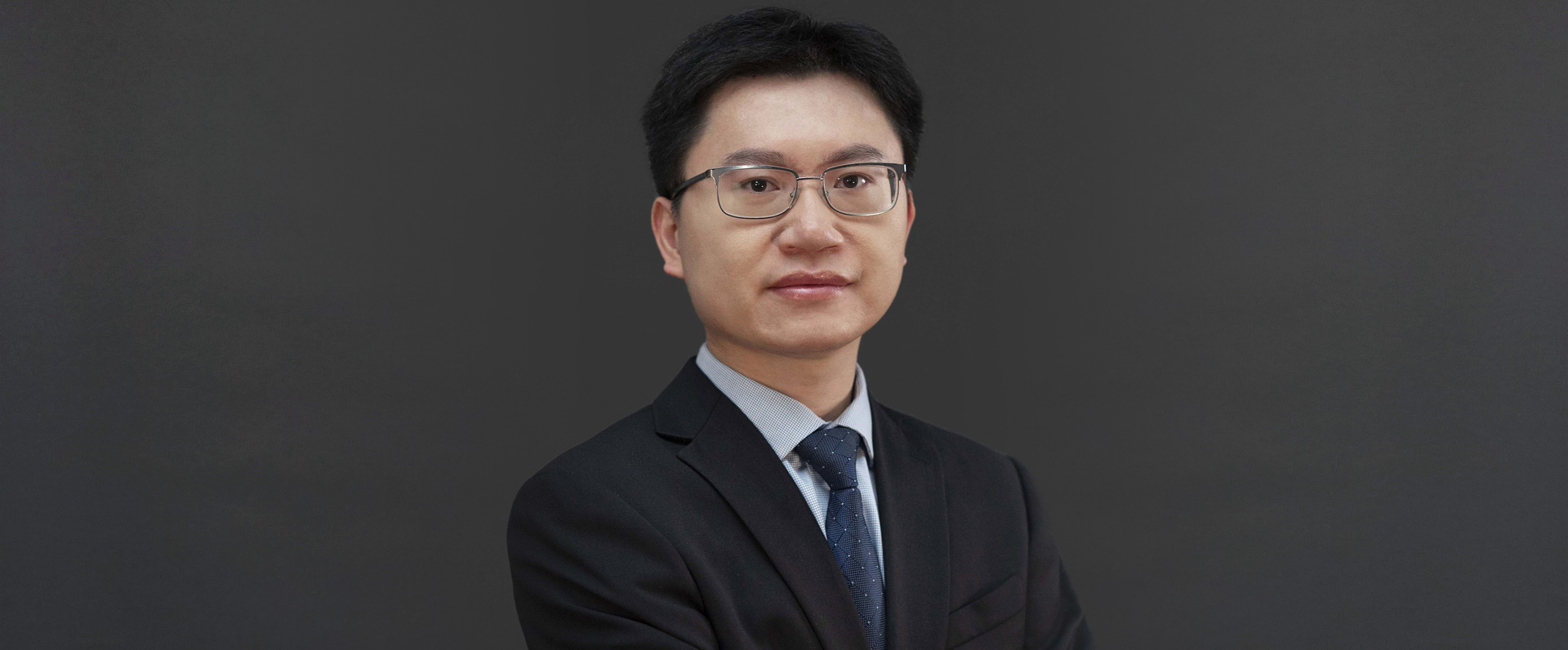
Hai Shu
Assistant Professor of Biostatistics
-
Professional overview
-
Dr. Hai Shu is an Assistant Professor in the Department of Biostatistics at New York University. He earned a Ph.D. in Biostatistics from University of Michigan and a B.S. in Information and Computational Science from Harbin Institute of Technology in China.
His research interests include high-dimensional data analysis (esp. data integration), machine/deep learning, medical image analysis (e.g., PET, MRI, Mammography), and their applications in Alzheimer’s disease, brain tumors, breast cancer, etc. He has published relevant papers in top-tier journals and conference, such as The Annals of Statistics, Journal of the American Statistical Association, Biometrics, and AAAI Conference on Artificial Intelligence. He has also served as a reviewer on related topics for Journal of the American Statistical Association, Statistica Sinica, International Joint Conference on Artificial Intelligence, etc.
Prior to joining NYU, Dr. Hai Shu was a Postdoctoral Fellow in the Department of Biostatistics at The University of Texas MD Anderson Cancer Center.
View Dr. Hai Shu's website at https://wp.nyu.edu/haishu
-
Education
-
Postdoctoral Fellow, Department of Biostatistics, The University of Texas MD Anderson Cancer Center, USAPh.D. in Biostatistics, Department of Biostatistics, University of Michigan, Ann Arbor, USAM.S. in Biostatistics, Department of Biostatistics, University of Michigan, Ann Arbor, USAB.S. in Information and Computational Science, Department of Mathematics, Harbin Institute of Technology (哈尔滨工业大学), China
-
Areas of research and study
-
Alzheimer’s diseaseBrain tumorsBreast cancerDeep learningHigh-dimensional data analysis/integrationMachine learningMedical image analysisSpatial/temporal data analysis
-
Publications
Publications
NCCT-to-CECT synthesis with contrast-enhanced knowledge and anatomical perception for multi-organ segmentation in non-contrast CT images
Multi-Scale Tokens-Aware Transformer Network for Multi-Region and Multi-Sequence MR-to-CT Synthesis in a Single Model
A generic fundus image enhancement network boosted by frequency self-supervised representation learning
Cross-Task Feedback Fusion GAN for Joint MR-CT Synthesis and Segmentation of Target and Organs-at-Risk
QACL: Quartet attention aware closed-loop learning for abdominal MR-to-CT synthesis via simultaneous registration
United multi-task learning for abdominal contrast-enhanced CT synthesis through joint deformable registration
A Comparative Study of non-deep Learning, Deep Learning, and Ensemble Learning Methods for Sunspot Number Prediction
Big Data and Machine Learning in Oncology
CDPA: Common and distinctive pattern analysis between high-dimensional datasets
D-GCCA: Decomposition-based Generalized Canonical Correlation Analysis for Multi-view High-dimensional Data.
A deep learning approach to re-create raw full-field digital mammograms for breast density and texture analysis
(TS)2WM: Tumor Segmentation and Tract Statistics for Assessing White Matter Integrity with Applications to Glioblastoma Patients
D-CCA: A Decomposition-Based Canonical Correlation Analysis for High-Dimensional Datasets
Assessment of network module identification across complex diseases
Estimation of large covariance and precision matrices from temporally dependent observations
Multiple testing for neuroimaging via hidden Markov random field