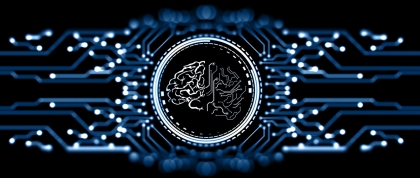
I’m pretty sure the laws of physics haven’t changed, but something about Chat GPT makes it feel like the world is moving faster and faster. These rapid changes lead me to consider how versions of these tools will soon become integral to daily life for us and our children. How do we effectively navigate these changes, taking into account both their potential solutions to complex human issues and their inherent biases and technological risks?
The speed at which things are moving propels a new vision for AI based on a public health framework, with the understanding that mitigating health inequities requires attention to their root causes, and that structural determinants can have a greater impact on the quality of life than healthcare spending or lifestyle choices alone. As a new twist on the old saying goes, “What's computed depends on who's computing it.”
To advance this vision of a public health-savvy AI, we recently launched an innovative community ecosystem approach to derive, catalog and utilize data representing the individual, community and other social/structural factors that shape our health. This community hub, created with our partners at Moi University in Kenya as a supplement to our NIH-funded NYU-Moi Data Science for Social Determinants Training Program, is an ecosystem of local biostatisticians and data science experts. As a first activity, this group is cataloging data from existing research or regionally representative surveys on the social and structural determinants of health. In a fruitful synergy, the group will also use their machine learning and data science expertise to generate new measures of social and structural factors that are unique to their region.
Elevating expertise from different places is crucial as ideas may travel globally, but they take shape locally. The more specific your data is to a population, the more you can create tailored solutions. For example, we know that discrimination can affect populations physically and emotionally, but how it’s measured in Nairobi will differ from how it’s measured in New York. These distinctions are critical when informing models to account for, analyze and implement interventions on social, built and structural environmental factors. Rightfully so, local experts are in the best position to identify, capture and model this data.
This community ecosystem approach also allows local populations to own their data and ensures the strength of privacy measures against technological risks. Local experts can help guide data literacy in their communities and inform collective decisions on what from their own data should be used and protected.
Our idea is that public health knowledge can shape technology, starting with who and what is represented in data — because data is the lifeblood of machine learning and artificial intelligence. Public health expertise with a local lens can also inform which problems are addressed with automated technologies like AI. As a public health community, it's time to seize this untapped innovation and proactively utilize our essential principles to drive the development of innovative and truly positively impactful technologies.
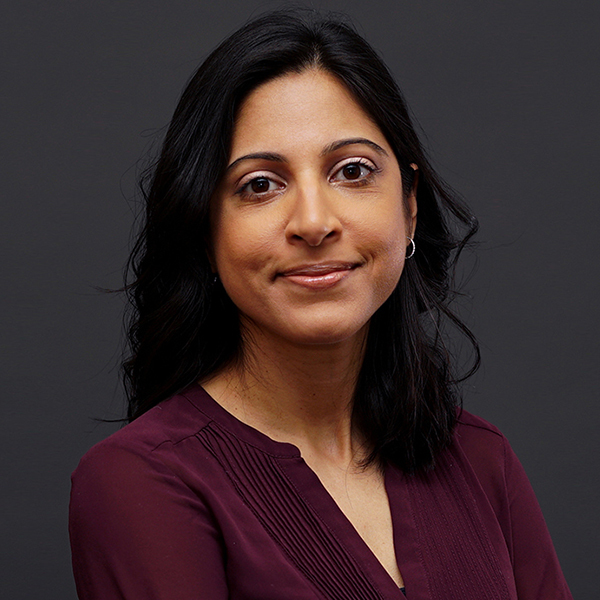
Rumi Chunara, PhD
Associate Professor of Biostatistics; Director of the Center for Health Data Science