Rumi Chunara
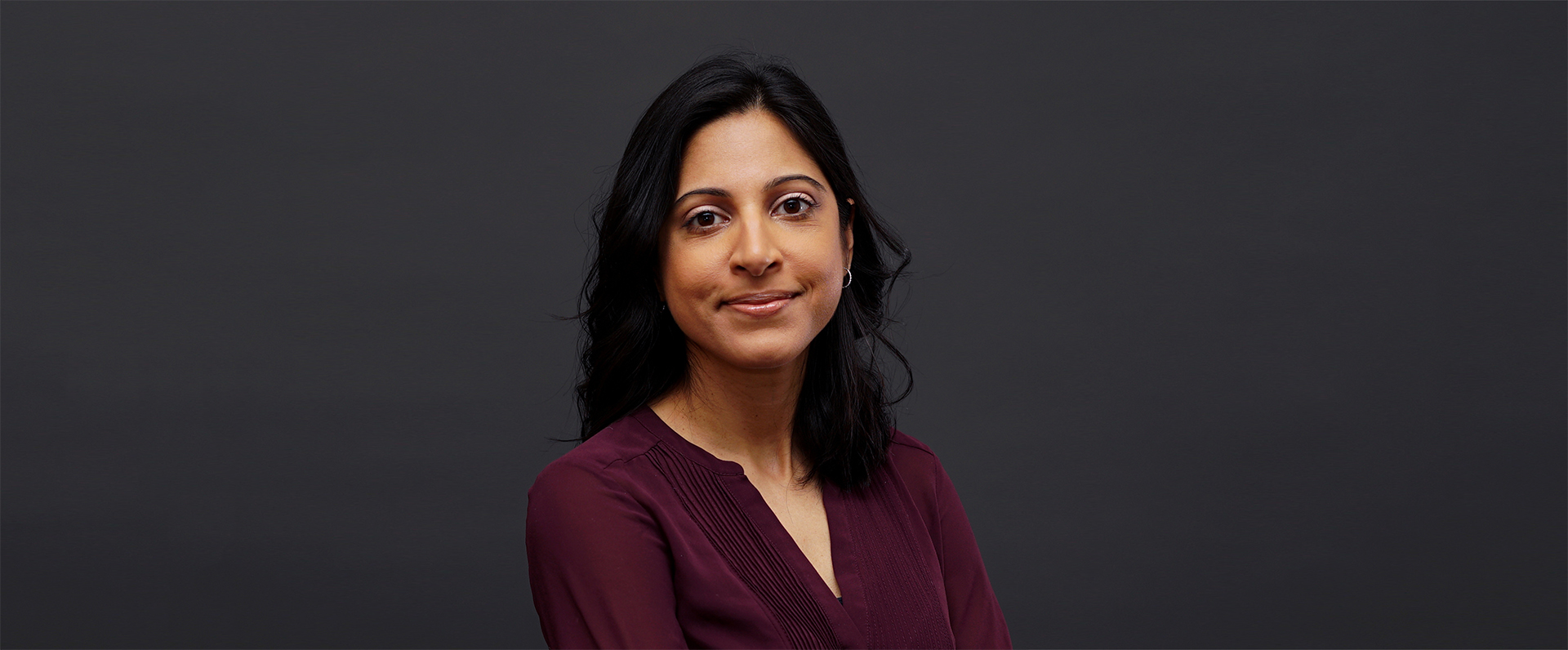
Rumi Chunara
Associate Professor of Biostatistics
Associate Professor of Computer Science and Engineering, Tandon
Director of Center for Health Data Science
-
Professional overview
-
The overarching goal of Dr. Rumi Chunara's research is to develop computational and statistical approaches for acquiring, integrating and using data to improve population-level public health. She focuses on the design and development of data mining and machine learning methods to address challenges related to data and goals of public health, as well as fairness and ethics in the design and use of data and algorithms embedded in social systems.
At NYU, Dr. Chunara also leads the Chunara Lab, which develops computational and statistical methods across data mining, natural language processing, spatio-temporal analyses and machine learning, to study population health. Previously, she was a Postdoctoral Fellow and Instructor at HealthMap and the Children's Hospital Informatics Program at Harvard Medical School. She completed her PhD at the Harvard-MIT Division of Health Sciences and Technology and BSc at Caltech.
-
Education
-
BS, Electrical Engineering (Honors), CaltechMS, Electrical Engineering and Computer Science, MITPhD, Medical and Electrical Engineering, MIT (Harvard-MIT Division of Health Sciences and Technology)
-
Honors and awards
-
Max Planck Sabbatical Award (2021)speaker at NSF Computer and Information Science and Engineering Directorate Career Proposal Writing Workshop (2020)Invited tutorial on Public Health and Machine Learning at ACM Conference on Health, Inference and Learning (2020)Keynote at Human Computation and Crowdsourcing (2019)Invited Speaker at Expert Group Meeting at United Nations Population Fund, Advances in Mobile Technologies for Data Collection Panel (2019)Keynote at ''Mapping the Equity Dimensions of Artificial Intelligence in Public Health'', University of Toronto (2019)Facebook Research Award (2019)Gates Foundation Grand Challenges Exploration Award (2019)NSF CAREER Award (2019)MIT Technology Review Top 35 Innovators Under 35 (2014)MIT Presidential Fellow (2004)
-
Areas of research and study
-
Health DisparitiesMachine learningSocial ComputingSocial Determinants of Health
-
Publications
Publications
Comparison of Social Media, Syndromic Surveillance, and Microbiologic Acute Respiratory Infection Data: Observational Study
Daughton, A. R., Chunara, R., & Paul, M. J. (n.d.).Publication year
2020Journal title
JMIR Public Health and SurveillanceVolume
6Issue
2AbstractBackground: Internet data can be used to improve infectious disease models. However, the representativeness and individual-level validity of internet-derived measures are largely unexplored as this requires ground truth data for study. Objective: This study sought to identify relationships between Web-based behaviors and/or conversation topics and health status using a ground truth, survey-based dataset. Methods: This study leveraged a unique dataset of self-reported surveys, microbiological laboratory tests, and social media data from the same individuals toward understanding the validity of individual-level constructs pertaining to influenza-like illness in social media data. Logistic regression models were used to identify illness in Twitter posts using user posting behaviors and topic model features extracted from users’ tweets. Results: Of 396 original study participants, only 81 met the inclusion criteria for this study. Of these participants’ tweets, we identified only two instances that were related to health and occurred within 2 weeks (before or after) of a survey indicating symptoms. It was not possible to predict when participants reported symptoms using features derived from topic models (area under the curve [AUC]=0.51; P=.38), though it was possible using behavior features, albeit with a very small effect size (AUC=0.53; P≤.001). Individual symptoms were also generally not predictable either. The study sample and a random sample from Twitter are predictably different on held-out data (AUC=0.67; P≤.001), meaning that the content posted by people who participated in this study was predictably different from that posted by random Twitter users. Individuals in the random sample and the GoViral sample used Twitter with similar frequencies (similar @ mentions, number of tweets, and number of retweets; AUC=0.50; P=.19). Conclusions: To our knowledge, this is the first instance of an attempt to use a ground truth dataset to validate infectious disease observations in social media data. The lack of signal, the lack of predictability among behaviors or topics, and the demonstrated volunteer bias in the study population are important findings for the large and growing body of disease surveillance using internet-sourced data.COVID-19 transforms health care through telemedicine: Evidence from the field
Mann, D. M., Chen, J., Chunara, R., Testa, P. A., & Nov, O. (n.d.).Publication year
2020Journal title
Journal of the American Medical Informatics AssociationVolume
27Issue
7Page(s)
1132-1135AbstractThis study provides data on the feasibility and impact of video-enabled telemedicine use among patients and providers and its impact on urgent and nonurgent healthcare delivery from one large health system (NYU Langone Health) at the epicenter of the coronavirus disease 2019 (COVID-19) outbreak in the United States. Between March 2nd and April 14th 2020, telemedicine visits increased from 102.4 daily to 801.6 daily. (683% increase) in urgent care after the system-wide expansion of virtual urgent care staff in response to COVID-19. Of all virtual visits post expansion, 56.2% and 17.6% urgent and nonurgent visits, respectively, were COVID-19-related. Telemedicine usage was highest by patients 20 to 44 years of age, particularly for urgent care. The COVID-19 pandemic has driven rapid expansion of telemedicine use for urgent care and nonurgent care visits beyond baseline periods. This reflects an important change in telemedicine that other institutions facing the COVID-19 pandemic should anticipate.Quantifying depression-related language on social media during the COVID-19 pandemic
Davis, B. D., McKnight, D. E., Teodorescu, D., Quan-Haase, A., Chunara, R., Fyshe, A., & Lizotte, D. J. (n.d.).Publication year
2020Journal title
International Journal of Population Data ScienceVolume
5Issue
4Page(s)
1716AbstractIntroduction The COVID-19 pandemic had clear impacts on mental health. Social media presents an opportunity for assessing mental health at the population level. Objectives 1) Identify and describe language used on social media that is associated with discourse about depression. 2) Describe the associations between identified language and COVID-19 incidence over time across several geographies. Methods We create a word embedding based on the posts in Reddit's/r/Depression and use this word embedding to train representations of active authors. We contrast these authors against a control group and extract keywords that capture differences between the two groups. We filter these keywords for face validity and to match character limits of an information retrieval system, Elasticsearch. We retrieve all geo-tagged posts on Twitter from April 2019 to June 2021 from Seattle, Sydney, Mumbai, and Toronto. The tweets are scored with BM25 using the keywords. We call this score rDD. We compare changes in average score over time with case counts from the pandemic's beginning through June 2021. Results We observe a pattern in rDD across all cities analyzed: There is an increase in rDD near the start of the pandemic which levels off over time. However, in Mumbai we also see an increase aligned with a second wave of cases. Conclusions Our results are concordant with other studies which indicate that the impact of the pandemic on mental health was highest initially and was followed by recovery, largely unchanged by subsequent waves. However, in the Mumbai data we observed a substantial rise in rDD with a large second wave. Our results indicate possible un-captured heterogeneity across geographies, and point to a need for a better understanding of this differential impact on mental health.Quantifying the localized relationship between vector containment activities and dengue incidence in a real-world setting: A spatial and time series modelling analysis based on geo-located data from Pakistan
Rehman, N. A., Salje, H., Kraemer, M. U., Subramanian, L., Saif, U., & Chunara, R. (n.d.).Publication year
2020Journal title
PLoS neglected tropical diseasesVolume
14Issue
5Page(s)
1-22AbstractIncreasing urbanization is having a profound effect on infectious disease risk, posing significant challenges for governments to allocate limited resources for their optimal control at a sub-city scale. With recent advances in data collection practices, empirical evidence about the efficacy of highly localized containment and intervention activities, which can lead to optimal deployment of resources, is possible. However, there are several challenges in analyzing data from such real-world observational settings. Using data on 3.9 million instances of seven dengue vector containment activities collected between 2012 and 2017, here we develop and assess two frameworks for understanding how the generation of new dengue cases changes in space and time with respect to application of different types of containment activities. Accounting for the non-random deployment of each containment activity in relation to dengue cases and other types of containment activities, as well as deployment of activities in different epidemiological contexts, results from both frameworks reinforce existing knowledge about the efficacy of containment activities aimed at the adult phase of the mosquito lifecycle. Results show a 10% (95% CI: 1–19%) and 20% reduction (95% CI: 4–34%) reduction in probability of a case occurring in 50 meters and 30 days of cases which had Indoor Residual Spraying (IRS) and fogging performed in the immediate vicinity, respectively, compared to cases of similar epidemiological context and which had no containment in their vicinity. Simultaneously, limitations due to the real-world nature of activity deployment are used to guide recommendations for future deployment of resources during outbreaks as well as data collection practices. Conclusions from this study will enable more robust and comprehensive analyses of localized containment activities in resource-scarce urban settings and lead to improved allocation of resources of government in an outbreak setting.Role of the built and online social environments on expression of dining on instagram
Mhasawade, V., Elghafari, A., Duncan, D. T., & Chunara, R. (n.d.).Publication year
2020Journal title
International journal of environmental research and public healthVolume
17Issue
3AbstractOnline social communities are becoming windows for learning more about the health of populations, through information about our health-related behaviors and outcomes from daily life. At the same time, just as public health data and theory has shown that aspects of the built environment can affect our health-related behaviors and outcomes, it is also possible that online social environments (e.g., posts and other attributes of our online social networks) can also shape facets of our life. Given the important role of the online environment in public health research and implications, factors which contribute to the generation of such data must be well understood. Here we study the role of the built and online social environments in the expression of dining on Instagram in Abu Dhabi; a ubiquitous social media platform, city with a vibrant dining culture, and a topic (food posts) which has been studied in relation to public health outcomes. Our study uses available data on user Instagram profiles and their Instagram networks, as well as the local food environment measured through the dining types (e.g., casual dining restaurants, food court restaurants, lounges etc.) by neighborhood. We find evidence that factors of the online social environment (profiles that post about dining versus profiles that do not post about dining) have different influences on the relationship between a user’s built environment and the social dining expression, with effects also varying by dining types in the environment and time of day. We examine the mechanism of the relationships via moderation and mediation analyses. Overall, this study provides evidence that the interplay of online and built environments depend on attributes of said environments and can also vary by time of day. We discuss implications of this synergy for precisely-targeting public health interventions, as well as on using online data for public health research.Using Digital Data to Protect and Promote the Most Vulnerable in the Fight Against COVID-19
Chunara, R., & Cook, S. H. (n.d.).Publication year
2020Journal title
Frontiers in Public HealthVolume
8Reports of the workshops held at the 2019 international AAAI conference on web and social media
Alburez-Gutierrez, D., Chandrasekharan, E., Chunara, R., Gil-Clavel, S., Hannak, A., Interdonato, R., Joseph, K., Kalimeri, K., Kairam, S., Malik, M. M., Mayer, K., Mejova, Y., Paolotti, D., & Zagheni, E. (n.d.).Publication year
2019Journal title
AI MagazineVolume
40Issue
4Page(s)
78-82Quantitative methods for measuring neighborhood characteristics in neighborhood health research
Duncan, D. T., Goedel, W. C., & Chunara, R. (n.d.). In Neighborhoods and Health (1–).Publication year
2018Page(s)
57-90Reports of the workshops held at the 2018 international AAAI conference on web and social media
An, J., Chunara, R., Crandall, D. J., Frajberg, D., French, M., Jansen, B. J., Kulshrestha, J., Mejova, Y., Romero, D. M., Salminen, J., Sharma, A., Sheth, A., Tan, C., Taylor, S. H., & Wijeratne, S. (n.d.).Publication year
2018Journal title
AI MagazineVolume
39Issue
4Page(s)
36-44Socio-spatial self-organizing maps: Using social media to assess relevant geographies for exposure to social processes
Relia, K., Akbari, M., Duncan, D., & Chunara, R. (n.d.).Publication year
2018Journal title
Proceedings of the ACM on Human-Computer InteractionVolume
2AbstractSocial media offers a unique window into attitudes like racism and homophobia, exposure to which are important, hard to measure and understudied social determinants of health. However, individual geo-located observations from social media are noisy and geographically inconsistent. Existing areas by which exposures are measured, like Zip codes, average over irrelevant administratively-defined boundaries. Hence, in order to enable studies of online social environmental measures like attitudes on social media and their possible relationship to health outcomes, first there is a need for a method to define the collective, underlying degree of social media attitudes by region. To address this, we create the Socio-spatial-Self organizing map, “SS-SOM” pipeline to best identify regions by their latent social attitude from Twitter posts. SS-SOMs use neural embedding for text-classification, and augment traditional SOMs to generate a controlled number of non-overlapping, topologically-constrained and topically-similar clusters. We find that not only are SS-SOMs robust to missing data, the exposure of a cohort of men who are susceptible to multiple racism and homophobia-linked health outcomes, changes by up to 42% using SS-SOM measures as compared to using Zip code-based measures.Tracking health seeking behavior during an Ebola outbreak via mobile phones and SMS
Feng, S., Grépin, K. A., & Chunara, R. (n.d.).Publication year
2018Journal title
npj Digital MedicineVolume
1Issue
1AbstractThe recent Ebola outbreak in West Africa was an exemplar for the need to rapidly measure population-level health-seeking behaviors, in order to understand healthcare utilization during emergency situations. Taking advantage of the high prevalence of mobile phones, we deployed a national SMS-poll and collected data about individual-level health and health-seeking behavior throughout the outbreak from 6694 individuals from March to June 2015 in Liberia. Using propensity score matching to generate balanced subsamples, we compared outcomes in our survey to those from a recent household survey (the 2013 Liberian Demographic Health Survey). We found that the matched subgroups had similar patterns of delivery location in aggregate, and utilizing data on the date of birth, we were able to show that facility-based deliveries were significantly decreased during, compared to after the outbreak (p < 0.05) consistent with findings from retrospective studies using healthcare-based data. Directly assessing behaviors from individuals via SMS also enabled the measurement of public and private sector facility utilization separately, which has been a challenge in other studies in countries including Liberia which rely mainly on government sources of data. In doing so, our data suggest that public facility-based deliveries returned to baseline values after the outbreak. Thus, we demonstrate that with the appropriate methodological approach to account for different population denominators, data sourced via mobile tools such as SMS polling could serve as an important low-cost complement to existing data collection strategies especially in situations where higher-frequency data than can be feasibly obtained through surveys is useful.Denominator Issues for Personally Generated Data in Population Health Monitoring
Chunara, R., Wisk, L. E., & Weitzman, E. R. (n.d.).Publication year
2017Journal title
American journal of preventive medicineVolume
52Issue
4Page(s)
549-553Determinants of participants' follow-up and characterization of representativeness in flu near you, a participatory disease surveillance system
Baltrusaitis, K., Santillana, M., Crawley, A. W., Chunara, R., Smolinski, M., & Brownstein, J. S. (n.d.).Publication year
2017Journal title
JMIR Public Health and SurveillanceVolume
3Issue
2AbstractBackground: Flu Near You (FNY) is an Internet-based participatory surveillance system in the United States and Canada that allows volunteers to report influenza-like symptoms using a brief weekly symptom report. Objective: Our objective was to evaluate the representativeness of the FNY population compared with the general population of the United States, explore the demographic and behavioral characteristics associated with FNY's high-participation users, and summarize results from a user survey of a cohort of FNY participants. Methods: We compared (1) the representativeness of sex and age groups of FNY participants during the 2014-2015 flu season versus the general US population and (2) the distribution of Human Development Index (HDI) scores of FNY participants versus that of the general US population. We analyzed associations between demographic and behavioral factors and the level of participant follow-up (ie, high vs low). Finally, descriptive statistics of responses from FNY's 2015 and 2016 end-of-season user surveys were calculated. Results: During the 2014-2015 influenza season, 47,234 unique participants had at least one FNY symptom report that was either self-reported (users) or submitted on their behalf (household members). The proportion of female FNY participants was significantly higher than that of the general US population (n=28,906, 61.2% vs 51.1%, P<.001). Although each age group was represented in the FNY population, the age distribution was significantly different from that of the US population (P<.001). Compared with the US population, FNY had a greater proportion of individuals with HDI >5.0, signaling that the FNY user distribution was more affluent and educated than the US population baseline. We found that high-participation use (ie, higher participation in follow-up symptom reports) was associated with sex (females were 25% less likely than men to be high-participation users), higher HDI, not reporting an influenza-like illness at the first symptom report, older age, and reporting for household members (all differences between high- and low-participation users P<.001). Approximately 10% of FNY users completed an additional survey at the end of the flu season that assessed detailed user characteristics (3217/33,324 in 2015; 4850/44,313 in 2016). Of these users, most identified as being either retired or employed in the health, education, and social services sectors and indicated that they achieved a bachelor's degree or higher. Conclusions: The representativeness of the FNY population and characteristics of its high-participation users are consistent with what has been observed in other Internet-based influenza surveillance systems. With targeted recruitment of underrepresented populations, FNY may improve as a complementary system to timely tracking of flu activity, especially in populations that do not seek medical attention and in areas with poor official surveillance data.Etiology of respiratory tract infections in the community and clinic in Ilorin, Nigeria
Kolawole, O., Oguntoye, M., Dam, T., & Chunara, R. (n.d.).Publication year
2017Journal title
BMC research notesVolume
10Issue
1Page(s)
712AbstractOBJECTIVE: Recognizing increasing interest in community disease surveillance globally, the goal of this study was to investigate whether respiratory viruses circulating in the community may be represented through clinical (hospital) surveillance in Nigeria.RESULTS: Children were selected via convenience sampling from communities and a tertiary care center (n = 91) during spring 2017 in Ilorin, Nigeria. Nasal swabs were collected and tested using polymerase chain reaction. The majority (79.1%) of subjects were under 6 years old, of whom 46 were infected (63.9%). A total of 33 of the 91 subjects had one or more respiratory tract virus; there were 10 cases of triple infection and 5 of quadruple. Parainfluenza virus 4, respiratory syncytial virus B and enterovirus were the most common viruses in the clinical sample; present in 93.8% (15/16) of clinical subjects, and 6.7% (5/75) of community subjects (significant difference, p < 0.001). Coronavirus OC43 was the most common virus detected in community members (13.3%, 10/75). A different strain, Coronavirus OC 229 E/NL63 was detected among subjects from the clinic (2/16) and not detected in the community. This pilot study provides evidence that data from the community can potentially represent different information than that sourced clinically, suggesting the need for community surveillance to enhance public health efforts and scientific understanding of respiratory infections.High-resolution temporal representations of alcohol and tobacco behaviors from social media data
Huang, T., Elghafari, A., Relia, K., & Chunara, R. (n.d.).Publication year
2017Journal title
Proceedings of the ACM on Human-Computer InteractionVolume
1AbstractUnderstanding tobacco- and alcohol-related behavioral patterns is critical for uncovering risk factors and potentially designing targeted social computing intervention systems. Given that we make choices multiple times per day, hourly and daily patterns are critical for better understanding behaviors. Here, we combine natural language processing, machine learning and time series analyses to assess Twitter activity specifically related to alcohol and tobacco consumption and their sub-daily, daily and weekly cycles. Twitter self-reports of alcohol and tobacco use are compared to other data streams available at similar temporal resolution. We assess if discussion of drinking by inferred underage versus legal age people or discussion of use of different types of tobacco products can be differentiated using these temporal patterns. We find that time and frequency domain representations of behaviors on social media can provide meaningful and unique insights, and we discuss the types of behaviors for which the approach may be most useful.Network inference from multimodal data: A review of approaches from infectious disease transmission
Ray, B., Ghedin, E., & Chunara, R. (n.d.).Publication year
2016Journal title
Journal of Biomedical InformaticsVolume
64Page(s)
44-54AbstractNetworks inference problems are commonly found in multiple biomedical subfields such as genomics, metagenomics, neuroscience, and epidemiology. Networks are useful for representing a wide range of complex interactions ranging from those between molecular biomarkers, neurons, and microbial communities, to those found in human or animal populations. Recent technological advances have resulted in an increasing amount of healthcare data in multiple modalities, increasing the preponderance of network inference problems. Multi-domain data can now be used to improve the robustness and reliability of recovered networks from unimodal data. For infectious diseases in particular, there is a body of knowledge that has been focused on combining multiple pieces of linked information. Combining or analyzing disparate modalities in concert has demonstrated greater insight into disease transmission than could be obtained from any single modality in isolation. This has been particularly helpful in understanding incidence and transmission at early stages of infections that have pandemic potential. Novel pieces of linked information in the form of spatial, temporal, and other covariates including high-throughput sequence data, clinical visits, social network information, pharmaceutical prescriptions, and clinical symptoms (reported as free-text data) also encourage further investigation of these methods. The purpose of this review is to provide an in-depth analysis of multimodal infectious disease transmission network inference methods with a specific focus on Bayesian inference. We focus on analytical Bayesian inference-based methods as this enables recovering multiple parameters simultaneously, for example, not just the disease transmission network, but also parameters of epidemic dynamics. Our review studies their assumptions, key inference parameters and limitations, and ultimately provides insights about improving future network inference methods in multiple applications.Characterizing sleep issues using Twitter
McIver, D. J., Hawkins, J. B., Chunara, R., Chatterjee, A. K., Bhandari, A., Fitzgerald, T. P., Jain, S. H., & Brownstein, J. S. (n.d.).Publication year
2015Journal title
Journal of medical Internet researchVolume
17Issue
6Page(s)
e140AbstractBackground: Sleep issues such as insomnia affect over 50 million Americans and can lead to serious health problems, including depression and obesity, and can increase risk of injury. Social media platforms such as Twitter offer exciting potential for their use in studying and identifying both diseases and social phenomenon. Objective: Our aim was to determine whether social media can be used as a method to conduct research focusing on sleep issues. Methods: Twitter posts were collected and curated to determine whether a user exhibited signs of sleep issues based on the presence of several keywords in tweets such as insomnia, "can't sleep", Ambien, and others. Users whose tweets contain any of the keywords were designated as having self-identified sleep issues (sleep group). Users who did not have self-identified sleep issues (non-sleep group) were selected from tweets that did not contain pre-defined words or phrases used as a proxy for sleep issues. Results: User data such as number of tweets, friends, followers, and location were collected, as well as the time and date of tweets. Additionally, the sentiment of each tweet and average sentiment of each user were determined to investigate differences between non-sleep and sleep groups. It was found that sleep group users were significantly less active on Twitter (P=.04), had fewer friends (P<.001), and fewer followers (P<.001) compared to others, after adjusting for the length of time each user's account has been active. Sleep group users were more active during typical sleeping hours than others, which may suggest they were having difficulty sleeping. Sleep group users also had significantly lower sentiment in their tweets (P<.001), indicating a possible relationship between sleep and pyschosocial issues. Conclusions: We have demonstrated a novel method for studying sleep issues that allows for fast, cost-effective, and customizable data to be gathered.Estimating influenza attack rates in the United States using a participatory cohort
Chunara, R., Goldstein, E., Patterson-Lomba, O., & Brownstein, J. S. (n.d.).Publication year
2015Journal title
Scientific reportsVolume
5AbstractWe considered how participatory syndromic surveillance data can be used to estimate influenza attack rates during the 2012-2013 and 2013-2014 seasons in the United States. Our inference is based on assessing the difference in the rates of self-reported influenza-like illness (ILI, defined as presence of fever and cough/sore throat) among the survey participants during periods of active vs. low influenza circulation as well as estimating the probability of self-reported ILI for influenza cases. Here, we combined Flu Near You data with additional sources (Hong Kong household studies of symptoms of influenza cases and the U.S. Centers for Disease Control and Prevention estimates of vaccine coverage and effectiveness) to estimate influenza attack rates. The estimated influenza attack rate for the early vaccinated Flu Near You members (vaccination reported by week 45) aged 20-64 between calendar weeks 47-12 was 14.7%(95% CI(5.9%,24.1%)) for the 2012-2013 season and 3.6%(â '3.3%,10.3%) for the 2013-2014 season. The corresponding rates for the US population aged 20-64 were 30.5% (4.4%, 49.3%) in 2012-2013 and 7.1%(-5.1%, 32.5%) in 2013-2014. The attack rates in women and men were similar each season. Our findings demonstrate that participatory syndromic surveillance data can be used to gauge influenza attack rates during future influenza seasons.Flu near you: Crowdsourced symptom reporting spanning 2 influenza seasons
Smolinski, M. S., Crawley, A. W., Baltrusaitis, K., Chunara, R., Olsen, J. M., Wójcik, O., Santillana, M., Nguyen, A., & Brownstein, J. S. (n.d.).Publication year
2015Journal title
American journal of public healthVolume
105Issue
10Page(s)
2124-2130AbstractObjectives. We summarized Flu Near You (FNY) data from the 2012?2013 and 2013?2014 influenza seasons in the United States. Methods. FNY collects limited demographic characteristic information upon registration, and prompts users each Monday to report symptoms of influenzalike illness (ILI) experienced during the previous week. We calculated the descriptive statistics and rates of ILI for the 2012?2013 and 2013?2014 seasons. We compared raw and noise-filtered ILI rates with ILI rates from the Centers for Disease Control and Prevention ILINet surveillance system. Results. More than 61 000 participants submitted at least 1 report during the 2012?2013 season, totaling 327 773 reports. Nearly 40 000 participants submitted at least 1 report during the 2013?2014 season, totaling 336 933 reports. Rates of ILI as reported by FNY tracked closely with ILINet in both timing and magnitude. Conclusions. With increased participation, FNY has the potential to serve as a viable complement to existing outpatient, hospital-based, and laboratory surveillance systems. Although many established systems have the benefits of specificity and credibility, participatory systems offer advantages in the areas of speed, sensitivity, and scalability.Surveillance of acute respiratory infections using community-submitted symptoms and specimens for molecular diagnostic testing
Goff, J., Rowe, A., Brownstein, J. S., & Chunara, R. (n.d.).Publication year
2015Journal title
PLoS CurrentsVolume
7AbstractParticipatory systems for surveillance of acute respiratory infection give real-time information about infections circulating in the community, yet to-date are limited to self-reported syndromic information only and lacking methods of linking symptom reports to infection types. We developed the GoViral platform to evaluate whether a cohort of lay volunteers could, and would find it useful to, contribute self-reported symptoms online and to compare specimen types for self-collected diagnostic information of sufficient quality for respiratory infection surveillance. Volunteers were recruited, given a kit (collection materials and customized instructions), instructed to report their symptoms weekly, and when sick with cold or flu-like symptoms, requested to collect specimens (saliva and nasal swab). We compared specimen types for respiratory virus detection sensitivity (via polymerase-chain-reaction) and ease of collection. Participants were surveyed to determine receptivity to participating when sick, to receiving information on the type of pathogen causing their infection and types circulating near them. Between December 1 2013 and March 1 2014, 295 participants enrolled in the study and received a kit. Of those who reported symptoms, half (71) collected and sent specimens for analysis. Participants submitted kits on average 2.30 days (95 CI: 1.65 to 2.96) after symptoms began. We found good concordance between nasal and saliva specimens for multiple pathogens, with few discrepancies. Individuals report that saliva collection is easiest and report that receiving information about what pathogen they, and those near them, have is valued and can shape public health behaviors. Community-submitted specimens can be used for the detection of acute respiratory infection with individuals showing receptivity for participating and interest in a real-time picture of respiratory pathogens near them.A case study of the New York City 2012-2013 influenza season with daily geocoded Twitter data from temporal and spatiotemporal perspectives
Nagar, R., Yuan, Q., Freifeld, C. C., Santillana, M., Nojima, A., Chunara, R., & Brownstein, J. S. (n.d.).Publication year
2014Journal title
Journal of medical Internet researchVolume
16Issue
10Page(s)
e236AbstractBackground: Twitter has shown some usefulness in predicting influenza cases on a weekly basis in multiple countries and on different geographic scales. Recently, Broniatowski and colleagues suggested Twitter's relevance at the city-level for New York City. Here, we look to dive deeper into the case of New York City by analyzing daily Twitter data from temporal and spatiotemporal perspectives. Also, through manual coding of all tweets, we look to gain qualitative insights that can help direct future automated searches. Objective: The intent of the study was first to validate the temporal predictive strength of daily Twitter data for influenza-like illness emergency department (ILI-ED) visits during the New York City 2012-2013 influenza season against other available and established datasets (Google search query, or GSQ), and second, to examine the spatial distribution and the spread of geocoded tweets as proxies for potential cases. Methods: From the Twitter Streaming API, 2972 tweets were collected in the New York City region matching the keywords "flu", "influenza", "gripe", and "high fever". The tweets were categorized according to the scheme developed by Lamb et al. A new fourth category was added as an evaluator guess for the probability of the subject(s) being sick to account for strength of confidence in the validity of the statement. Temporal correlations were made for tweets against daily ILI-ED visits and daily GSQ volume. The best models were used for linear regression for forecasting ILI visits. A weighted, retrospective Poisson model with SaTScan software (n=1484), and vector map were used for spatiotemporal analysis. Results: Infection-related tweets (R=.763) correlated better than GSQ time series (R=.683) for the same keywords and had a lower mean average percent error (8.4 vs 11.8) for ILI-ED visit prediction in January, the most volatile month of flu. SaTScan identified primary outbreak cluster of high-probability infection tweets with a 2.74 relative risk ratio compared to medium-probability infection tweets at P=.001 in Northern Brooklyn, in a radius that includes Barclay's Center and the Atlantic Avenue Terminal. Conclusions: While others have looked at weekly regional tweets, this study is the first to stress test Twitter for daily city-level data for New York City. Extraction of personal testimonies of infection-related tweets suggests Twitter's strength both qualitatively and quantitatively for ILI-ED prediction compared to alternative daily datasets mixed with awareness-based data such as GSQ. Additionally, granular Twitter data provide important spatiotemporal insights. A tweet vector-map may be useful for visualization of city-level spread when local gold standard data are otherwise unavailable.Public health for the people: Participatory infectious disease surveillance in the digital age
Wójcik, O. P., Brownstein, J. S., Chunara, R., & Johansson, M. A. (n.d.).Publication year
2014Journal title
Emerging Themes in EpidemiologyVolume
11Issue
1AbstractThe 21st century has seen the rise of Internet-based participatory surveillance systems for infectious diseases. These systems capture voluntarily submitted symptom data from the general public and can aggregate and communicate that data in near real-time. We reviewed participatory surveillance systems currently running in 13 different countries. These systems have a growing evidence base showing a high degree of accuracy and increased sensitivity and timeliness relative to traditional healthcare-based systems. They have also proven useful for assessing risk factors, vaccine effectiveness, and patterns of healthcare utilization while being less expensive, more flexible, and more scalable than traditional systems. Nonetheless, they present important challenges including biases associated with the population that chooses to participate, difficulty in adjusting for confounders, and limited specificity because of reliance only on syndromic definitions of disease limits. Overall, participatory disease surveillance data provides unique disease information that is not available through traditional surveillance sources.Assessing the Online Social Environment for Surveillance of Obesity Prevalence
Chunara, R., Bouton, L., Ayers, J. W., & Brownstein, J. S. (n.d.).Publication year
2013Journal title
PloS oneVolume
8Issue
4AbstractBackground:Understanding the social environmental around obesity has been limited by available data. One promising approach used to bridge similar gaps elsewhere is to use passively generated digital data.Purpose:This article explores the relationship between online social environment via web-based social networks and population obesity prevalence.Methods:We performed a cross-sectional study using linear regression and cross validation to measure the relationship and predictive performance of user interests on the online social network Facebook to obesity prevalence in metros across the United States of America (USA) and neighborhoods within New York City (NYC). The outcomes, proportion of obese and/or overweight population in USA metros and NYC neighborhoods, were obtained via the Centers for Disease Control and Prevention Behavioral Risk Factor Surveillance and NYC EpiQuery systems. Predictors were geographically specific proportion of users with activity-related and sedentary-related interests on Facebook.Results:Higher proportion of the population with activity-related interests on Facebook was associated with a significant 12.0% (95% Confidence Interval (CI) 11.9 to 12.1) lower predicted prevalence of obese and/or overweight people across USA metros and 7.2% (95% CI: 6.8 to 7.7) across NYC neighborhoods. Conversely, greater proportion of the population with interest in television was associated with higher prevalence of obese and/or overweight people of 3.9% (95% CI: 3.7 to 4.0) (USA) and 27.5% (95% CI: 27.1 to 27.9, significant) (NYC). For activity-interests and national obesity outcomes, the average root mean square prediction error from 10-fold cross validation was comparable to the average root mean square error of a model developed using the entire data set.Conclusions:Activity-related interests across the USA and sedentary-related interests across NYC were significantly associated with obesity prevalence. Further research is needed to understand how the online social environment relates to health outcomes and how it can be used to identify or target interventions.Monitoring Influenza Epidemics in China with Search Query from Baidu
Yuan, Q., Nsoesie, E. O., Lv, B., Peng, G., Chunara, R., & Brownstein, J. S. (n.d.).Publication year
2013Journal title
PloS oneVolume
8Issue
5AbstractSeveral approaches have been proposed for near real-time detection and prediction of the spread of influenza. These include search query data for influenza-related terms, which has been explored as a tool for augmenting traditional surveillance methods. In this paper, we present a method that uses Internet search query data from Baidu to model and monitor influenza activity in China. The objectives of the study are to present a comprehensive technique for: (i) keyword selection, (ii) keyword filtering, (iii) index composition and (iv) modeling and detection of influenza activity in China. Sequential time-series for the selected composite keyword index is significantly correlated with Chinese influenza case data. In addition, one-month ahead prediction of influenza cases for the first eight months of 2012 has a mean absolute percent error less than 11%. To our knowledge, this is the first study on the use of search query data from Baidu in conjunction with this approach for estimation of influenza activity in China.Twitter as a Sentinel in Emergency Situations: Lessons from the Boston Marathon Explosions
Cassa, C. A., Chunara, R., Mandl, K., & Brownstein, J. S. (n.d.).Publication year
2013Journal title
PLoS CurrentsAbstractImmediately following the Boston Marathon attacks, individuals near the scene posted a deluge of data to social media sites. Previous work has shown that these data can be leveraged to provide rapid insight during natural disasters, disease outbreaks and ongoing conflicts that can assist in the public health and medical response. Here, we examine and discuss the social media messages posted immediately after and around the Boston Marathon bombings, and find that specific keywords appear frequently prior to official public safety and news media reports. Individuals immediately adjacent to the explosions posted messages within minutes via Twitter which identify the location and specifics of events, demonstrating a role for social media in the early recognition and characterization of emergency events. *Christopher Cassa and Rumi Chunara contributed equally to this work.