Yang Feng
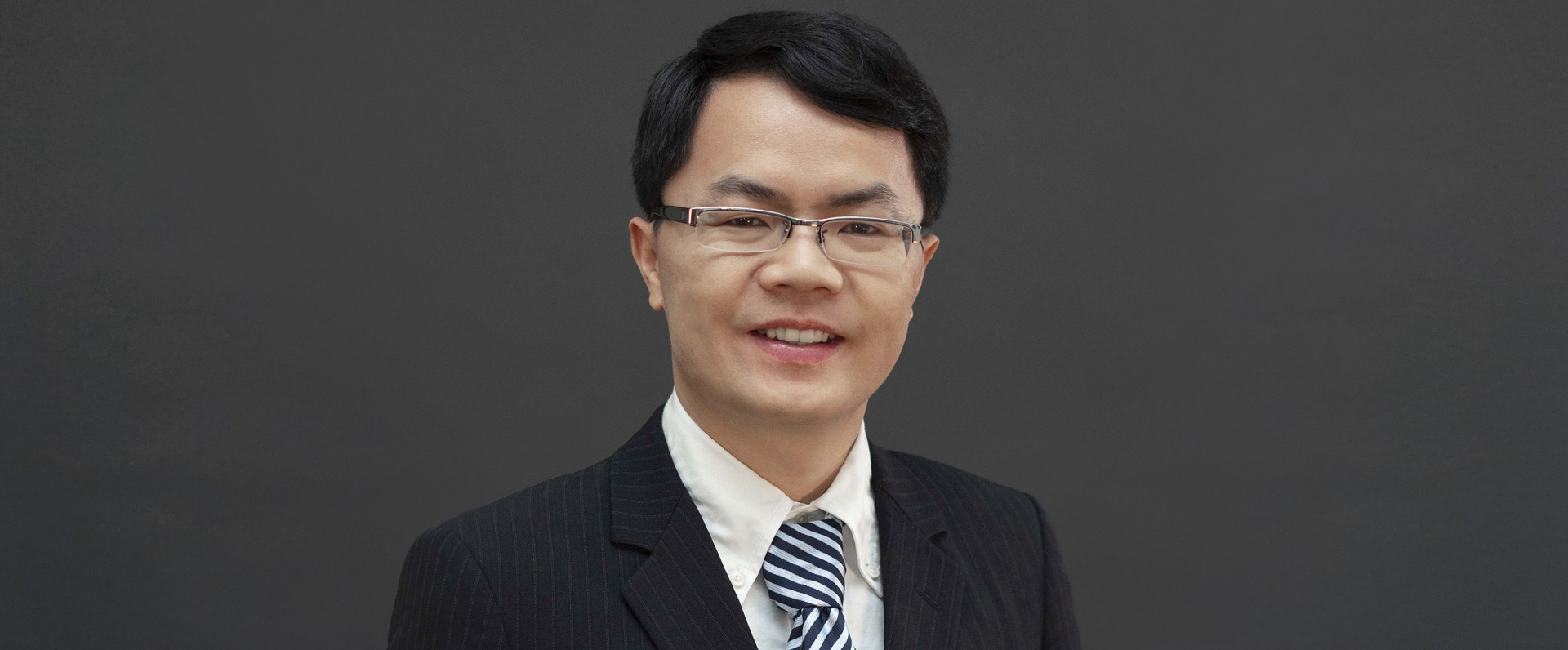
Professor of Biostatistics
-
Professional overview
-
Yang Feng is a Professor and Ph.D. Program Director of Biostatistics in the School of Global Public Health and an affiliate faculty in the Center for Data Science at New York University. He obtained his Ph.D. in Operations Research at Princeton University in 2010.
Feng's research interests encompass the theoretical and methodological aspects of machine learning, high-dimensional statistics, social network models, and nonparametric statistics, leading to a wealth of practical applications, including Alzheimer's disease, cancer classification, and electronic health records. His research has been funded by multiple grants from the National Institutes of Health (NIH) and the National Science Foundation (NSF), notably the NSF CAREER Award.
He is currently an Associate Editor for the Journal of the American Statistical Association (JASA), the Journal of Business & Economic Statistics (JBES), Journal of Computational & Graphical Statistics (JCGS), and the Annals of Applied Statistics (AoAS). His professional recognitions include being named a fellow of the American Statistical Association (ASA) and the Institute of Mathematical Statistics (IMS), as well as an elected member of the International Statistical Institute (ISI).
Please visit Dr. Yang Feng's website and Google Scholar page from more information.
-
Education
-
B.S. in Mathematics, University of Science and Technology of China, Hefei, ChinaPh.D. in Operations Research, Princeton University, Princeton, NJ
-
Areas of research and study
-
BioinformaticsBiostatisticsHigh-dimensional data analysis/integrationMachine learningModeling Social and Behavioral DynamicsNonparametric statistics
-
Publications
Publications
DDAC-SpAM: A Distributed Algorithm for Fitting High-dimensional Sparse Additive Models with Feature Division and Decorrelation
He, Y., Wu, R., Zhou, Y., & Feng, Y. (n.d.).Publication year
2024Journal title
Journal of the American Statistical AssociationVolume
119Issue
547Page(s)
1933-1944AbstractAbstract– Distributed statistical learning has become a popular technique for large-scale data analysis. Most existing work in this area focuses on dividing the observations, but we propose a new algorithm, DDAC-SpAM, which divides the features under a high-dimensional sparse additive model. Our approach involves three steps: divide, decorrelate, and conquer. The decorrelation operation enables each local estimator to recover the sparsity pattern for each additive component without imposing strict constraints on the correlation structure among variables. The effectiveness and efficiency of the proposed algorithm are demonstrated through theoretical analysis and empirical results on both synthetic and real data. The theoretical results include both the consistent sparsity pattern recovery as well as statistical inference for each additive functional component. Our approach provides a practical solution for fitting sparse additive models, with promising applications in a wide range of domains. Supplementary materials for this article are available online.Machine collaboration
Liu, Q., & Feng, Y. (n.d.).Publication year
2024Journal title
StatVolume
13Issue
1AbstractWe propose a new ensemble framework for supervised learning, called machine collaboration (MaC), using a collection of possibly heterogeneous base learning methods (hereafter, base machines) for prediction tasks. Unlike bagging/stacking (a parallel and independent framework) and boosting (a sequential and top-down framework), MaC is a type of circular and recursive learning framework. The circular and recursive nature helps the base machines to transfer information circularly and update their structures and parameters accordingly. The theoretical result on the risk bound of the estimator from MaC reveals that the circular and recursive feature can help MaC reduce risk via a parsimonious ensemble. We conduct extensive experiments on MaC using both simulated data and 119 benchmark real datasets. The results demonstrate that in most cases, MaC performs significantly better than several other state-of-the-art methods, including classification and regression trees, neural networks, stacking, and boosting.PCABM: Pairwise Covariates-Adjusted Block Model for Community Detection
Huang, S., Sun, J., & Feng, Y. (n.d.).Publication year
2024Journal title
Journal of the American Statistical AssociationVolume
119Issue
547Page(s)
2092-2104AbstractOne of the most fundamental problems in network study is community detection. The stochastic block model (SBM) is a widely used model, and various estimation methods have been developed with their community detection consistency results unveiled. However, the SBM is restricted by the strong assumption that all nodes in the same community are stochastically equivalent, which may not be suitable for practical applications. We introduce a pairwise covariates-adjusted stochastic block model (PCABM), a generalization of SBM that incorporates pairwise covariate information. We study the maximum likelihood estimators of the coefficients for the covariates as well as the community assignments, and show they are consistent under suitable sparsity conditions. Spectral clustering with adjustment (SCWA) is introduced to efficiently solve PCABM. Under certain conditions, we derive the error bound of community detection for SCWA and show that it is community detection consistent. In addition, we investigate model selection in terms of the number of communities and feature selection for the pairwise covariates, and propose two corresponding algorithms. PCABM compares favorably with the SBM or degree-corrected stochastic block model (DCBM) under a wide range of simulated and real networks when covariate information is accessible. Supplementary materials for this article are available online.Prognostic value of DNA methylation subclassification, aneuploidy, and CDKN2A/B homozygous deletion in predicting clinical outcome of IDH mutant astrocytomas
Galbraith, K., Garcia, M., Wei, S., Chen, A., Schroff, C., Serrano, J., Pacione, D., Placantonakis, D. G., William, C. M., Faustin, A., Zagzag, D., Barbaro, M., Del Pilar Guillermo Prieto Eibl, M., Shirahata, M., Reuss, D., Tran, Q. T., Alom, Z., Von Deimling, A., Orr, B. A., … Snuderl, M. (n.d.).Publication year
2024Journal title
Neuro-OncologyVolume
26Issue
6Page(s)
1042-1051AbstractBackground. Isocitrate dehydrogenase (IDH) mutant astrocytoma grading, until recently, has been entirely based on morphology. The 5th edition of the Central Nervous System World Health Organization (WHO) introduces CDKN2A/B homozygous deletion as a biomarker of grade 4. We sought to investigate the prognostic impact of DNA methylation-derived molecular biomarkers for IDH mutant astrocytoma. Methods. We analyzed 98 IDH mutant astrocytomas diagnosed at NYU Langone Health between 2014 and 2022. We reviewed DNA methylation subclass, CDKN2A/B homozygous deletion, and ploidy and correlated molecular biomarkers with histological grade, progression free (PFS), and overall (OS) survival. Findings were confirmed using 2 independent validation cohorts. Results. There was no significant difference in OS or PFS when stratified by histologic WHO grade alone, copy number complexity, or extent of resection. OS was significantly different when patients were stratified either by CDKN2A/B homozygous deletion or by DNA methylation subclass (P value = .0286 and .0016, respectively). None of the molecular biomarkers were associated with significantly better PFS, although DNA methylation classification showed a trend (P value = .0534). Conclusions. The current WHO recognized grading criteria for IDH mutant astrocytomas show limited prognostic value. Stratification based on DNA methylation shows superior prognostic value for OS.A flexible quasi-likelihood model for microbiome abundance count data
Shi, Y., Li, H., Wang, C., Chen, J., Jiang, H., Shih, Y. C. T., Zhang, H., Song, Y., Feng, Y., & Liu, L. (n.d.).Publication year
2023Journal title
Statistics in MedicineVolume
42Issue
25Page(s)
4632-4643AbstractIn this article, we present a flexible model for microbiome count data. We consider a quasi-likelihood framework, in which we do not make any assumptions on the distribution of the microbiome count except that its variance is an unknown but smooth function of the mean. By comparing our model to the negative binomial generalized linear model (GLM) and Poisson GLM in simulation studies, we show that our flexible quasi-likelihood method yields valid inferential results. Using a real microbiome study, we demonstrate the utility of our method by examining the relationship between adenomas and microbiota. We also provide an R package “fql” for the application of our method.Comments on: Statistical inference and large-scale multiple testing for high-dimensional regression models
Tian, Y., & Feng, Y. (n.d.).Publication year
2023Journal title
TestVolume
32Issue
4Page(s)
1172-1176RaSE: A Variable Screening Framework via Random Subspace Ensembles
Tian, Y., & Feng, Y. (n.d.).Publication year
2023Journal title
Journal of the American Statistical AssociationVolume
118Issue
541Page(s)
457-468AbstractVariable screening methods have been shown to be effective in dimension reduction under the ultra-high dimensional setting. Most existing screening methods are designed to rank the predictors according to their individual contributions to the response. As a result, variables that are marginally independent but jointly dependent with the response could be missed. In this work, we propose a new framework for variable screening, random subspace ensemble (RaSE), which works by evaluating the quality of random subspaces that may cover multiple predictors. This new screening framework can be naturally combined with any subspace evaluation criterion, which leads to an array of screening methods. The framework is capable to identify signals with no marginal effect or with high-order interaction effects. It is shown to enjoy the sure screening property and rank consistency. We also develop an iterative version of RaSE screening with theoretical support. Extensive simulation studies and real-data analysis show the effectiveness of the new screening framework.Simulation of New York City's Ventilator Allocation Guideline during the Spring 2020 COVID-19 Surge
Walsh, B. C., Zhu, J., Feng, Y., Berkowitz, K. A., Betensky, R. A., Nunnally, M. E., & Pradhan, D. R. (n.d.).Publication year
2023Journal title
JAMA network openVolume
6Issue
10Page(s)
E2336736AbstractImportance: The spring 2020 surge of COVID-19 unprecedentedly strained ventilator supply in New York City, with many hospitals nearly exhausting available ventilators and subsequently seriously considering enacting crisis standards of care and implementing New York State Ventilator Allocation Guidelines (NYVAG). However, there is little evidence as to how NYVAG would perform if implemented. Objectives: To evaluate the performance and potential improvement of NYVAG during a surge of patients with respect to the length of rationing, overall mortality, and worsening health disparities. Design, Setting, and Participants: This cohort study included intubated patients in a single health system in New York City from March through July 2020. A total of 20000 simulations were conducted of ventilator triage (10000 following NYVAG and 10000 following a proposed improved NYVAG) during a crisis period, defined as the point at which the prepandemic ventilator supply was 95% utilized. Exposures: The NYVAG protocol for triage ventilators. Main Outcomes and Measures: Comparison of observed survival rates with simulations of scenarios requiring NYVAG ventilator rationing. Results: The total cohort included 1671 patients; of these, 674 intubated patients (mean [SD] age, 63.7 [13.8] years; 465 male [69.9%]) were included in the crisis period, with 571 (84.7%) testing positive for COVID-19. Simulated ventilator rationing occurred for 163.9 patients over 15.0 days, 44.4% (95% CI, 38.3%-50.0%) of whom would have survived if provided a ventilator while only 34.8% (95% CI, 28.5%-40.0%) of those newly intubated patients receiving a reallocated ventilator survived. While triage categorization at the time of intubation exhibited partial prognostic differentiation, 94.8% of all ventilator rationing occurred after a time trial. Within this subset, 43.1% were intubated for 7 or more days with a favorable SOFA score that had not improved. An estimated 60.6% of these patients would have survived if sustained on a ventilator. Revising triage subcategorization, proposed improved NYVAG, would have improved this alarming ventilator allocation inefficiency (25.3% [95% CI, 22.1%-28.4%] of those selected for ventilator rationing would have survived if provided a ventilator). NYVAG ventilator rationing did not exacerbate existing health disparities. Conclusions and Relevance: In this cohort study of intubated patients experiencing simulated ventilator rationing during the apex of the New York City COVID-19 2020 surge, NYVAG diverted ventilators from patients with a higher chance of survival to those with a lower chance of survival. Future efforts should be focused on triage subcategorization, which improved this triage inefficiency, and ventilator rationing after a time trial, when most ventilator rationing occurred..Spectral Clustering via Adaptive Layer Aggregation for Multi-Layer Networks
Huang, S., Weng, H., & Feng, Y. (n.d.).Publication year
2023Journal title
Journal of Computational and Graphical StatisticsVolume
32Issue
3Page(s)
1170-1184AbstractOne of the fundamental problems in network analysis is detecting community structure in multi-layer networks, of which each layer represents one type of edge information among the nodes. We propose integrative spectral clustering approaches based on effective convex layer aggregations. Our aggregation methods are strongly motivated by a delicate asymptotic analysis of the spectral embedding of weighted adjacency matrices and the downstream k-means clustering, in a challenging regime where community detection consistency is impossible. In fact, the methods are shown to estimate the optimal convex aggregation, which minimizes the misclustering error under some specialized multi-layer network models. Our analysis further suggests that clustering using Gaussian mixture models is generally superior to the commonly used k-means in spectral clustering. Extensive numerical studies demonstrate that our adaptive aggregation techniques, together with Gaussian mixture model clustering, make the new spectral clustering remarkably competitive compared to several popularly used methods. Supplementary materials for this article are available online.Transfer Learning Under High-Dimensional Generalized Linear Models
Tian, Y., & Feng, Y. (n.d.).Publication year
2023Journal title
Journal of the American Statistical AssociationVolume
118Issue
544Page(s)
2684-2697AbstractIn this work, we study the transfer learning problem under high-dimensional generalized linear models (GLMs), which aim to improve the fit on target data by borrowing information from useful source data. Given which sources to transfer, we propose a transfer learning algorithm on GLM, and derive its (Formula presented.) -estimation error bounds as well as a bound for a prediction error measure. The theoretical analysis shows that when the target and sources are sufficiently close to each other, these bounds could be improved over those of the classical penalized estimator using only target data under mild conditions. When we don’t know which sources to transfer, an algorithm-free transferable source detection approach is introduced to detect informative sources. The detection consistency is proved under the high-dimensional GLM transfer learning setting. We also propose an algorithm to construct confidence intervals of each coefficient component, and the corresponding theories are provided. Extensive simulations and a real-data experiment verify the effectiveness of our algorithms. We implement the proposed GLM transfer learning algorithms in a new R package glmtrans, which is available on CRAN. Supplementary materials for this article are available online.Variable selection for high-dimensional generalized linear model with block-missing data
He, Y., Feng, Y., & Song, X. (n.d.).Publication year
2023Journal title
Scandinavian Journal of StatisticsVolume
50Issue
3Page(s)
1279-1297AbstractIn modern scientific research, multiblock missing data emerges with synthesizing information across multiple studies. However, existing imputation methods for handling block-wise missing data either focus on the single-block missing pattern or heavily rely on the model structure. In this study, we propose a single regression-based imputation algorithm for multiblock missing data. First, we conduct a sparse precision matrix estimation based on the structure of block-wise missing data. Second, we impute the missing blocks with their means conditional on the observed blocks. Theoretical results about variable selection and estimation consistency are established in the context of a generalized linear model. Moreover, simulation studies show that compared with existing methods, the proposed imputation procedure is robust to various missing mechanisms because of the good properties of regression imputation. An application to Alzheimer's Disease Neuroimaging Initiative data also confirms the superiority of our proposed method.A likelihood-ratio type test for stochastic block models with bounded degrees
Yuan, M., Feng, Y., & Shang, Z. (n.d.).Publication year
2022Journal title
Journal of Statistical Planning and InferenceVolume
219Page(s)
98-119AbstractA fundamental problem in network data analysis is to test Erdös–Rényi model [Formula presented] versus a bisection stochastic block model [Formula presented], where a,b>0 are constants that represent the expected degrees of the graphs and n denotes the number of nodes. This problem serves as the foundation of many other problems such as testing-based methods for determining the number of communities (Bickel and Sarkar, 2016; Lei, 2016) and community detection (Montanari and Sen, 2016). Existing work has been focusing on growing-degree regime a,b→∞ (Bickel and Sarkar, 2016; Lei, 2016; Montanari and Sen, 2016; Banerjee and Ma, 2017; Banerjee, 2018; Gao and Lafferty, 2017a,b) while leaving the bounded-degree regime untreated. In this paper, we propose a likelihood-ratio (LR) type procedure based on regularization to test stochastic block models with bounded degrees. We derive the limit distributions as power Poisson laws under both null and alternative hypotheses, based on which the limit power of the test is carefully analyzed. We also examine a Monte-Carlo method that partly resolves the computational cost issue. The proposed procedures are examined by both simulated and real-world data. The proof depends on a contiguity theory developed by Janson (1995).Association of hyperglycemia and molecular subclass on survival in IDH-wildtype glioblastoma
Liu, E. K., Vasudevaraja, V., Sviderskiy, V. O., Feng, Y., Tran, I., Serrano, J., Cordova, C., Kurz, S. C., Golfinos, J. G., Sulman, E. P., Orringer, D. A., Placantonakis, D., Possemato, R., & Snuderl, M. (n.d.).Publication year
2022Journal title
Neuro-Oncology AdvancesVolume
4Issue
1AbstractBackground: Hyperglycemia has been associated with worse survival in glioblastoma. Attempts to lower glucose yielded mixed responses which could be due to molecularly distinct GBM subclasses. Methods: Clinical, laboratory, and molecular data on 89 IDH-wt GBMs profiled by clinical next-generation sequencing and treated with Stupp protocol were reviewed. IDH-wt GBMs were sub-classified into RTK I (Proneural), RTK II (Classical) and Mesenchymal subtypes using whole-genome DNA methylation. Average glucose was calculated by time-weighting glucose measurements between diagnosis and last follow-up. Results: Patients were stratified into three groups using average glucose: tertile one (<100 mg/dL), tertile two (100-115 mg/dL), and tertile three (>115 mg/dL). Comparison across glucose tertiles revealed no differences in performance status (KPS), dexamethasone dose, MGMT methylation, or methylation subclass. Overall survival (OS) was not affected by methylation subclass (P =. 9) but decreased with higher glucose (P =. 015). Higher glucose tertiles were associated with poorer OS among RTK I (P =. 08) and mesenchymal tumors (P =. 05), but not RTK II (P =. 99). After controlling for age, KPS, dexamethasone, and MGMT status, glucose remained significantly associated with OS (aHR = 5.2, P =. 02). Methylation clustering did not identify unique signatures associated with high or low glucose levels. Metabolomic analysis of 23 tumors showed minimal variation across metabolites without differences between molecular subclasses. Conclusion: Higher average glucose values were associated with poorer OS in RTKI and Mesenchymal IDH-wt GBM, but not RTKII. There were no discernible epigenetic or metabolomic differences between tumors in different glucose environments, suggesting a potential survival benefit to lowering systemic glucose in selected molecular subtypes.Clinical, Pathological, and Molecular Characteristics of Diffuse Spinal Cord Gliomas
Garcia, M. R., Feng, Y., Vasudevaraja, V., Galbraith, K., Serrano, J., Thomas, C., Radmanesh, A., Hidalgo, E. T., Harter, D. H., Allen, J. C., Gardner, S. L., Osorio, D. S., William, C. M., Zagzag, D., Boué, D. R., & Snuderl, M. (n.d.).Publication year
2022Journal title
Journal of Neuropathology and Experimental NeurologyVolume
81Issue
11Page(s)
865-872AbstractDiffuse spinal cord gliomas (SCGs) are rare tumors associated with a high morbidity and mortality that affect both pediatric and adult populations. In this retrospective study, we sought to characterize the clinical, pathological, and molecular features of diffuse SCG in 22 patients with histological and molecular analyses. The median age of our cohort was 23.64 years (range 1-82) and the overall median survival was 397 days. K27M mutation was significantly more prevalent in males compared to females. Gross total resection and chemotherapy were associated with improved survival, compared to biopsy and no chemotherapy. While there was no association between tumor grade, K27M status (p = 0.366) or radiation (p = 0.772), and survival, males showed a trend toward shorter survival. K27M mutant tumors showed increased chromosomal instability and a distinct DNA methylation signature.Community detection with nodal information: Likelihood and its variational approximation
Weng, H., & Feng, Y. (n.d.).Publication year
2022Journal title
StatVolume
11Issue
1AbstractCommunity detection is one of the fundamental problems in the study of network data. Most existing community detection approaches only consider edge information as inputs, and the output could be suboptimal when nodal information is available. In such cases, it is desirable to leverage nodal information for the improvement of community detection accuracy. Towards this goal, we propose a flexible network model incorporating nodal information and develop likelihood-based inference methods. For the proposed methods, we establish favorable asymptotic properties as well as efficient algorithms for computation. Numerical experiments show the effectiveness of our methods in utilizing nodal information across a variety of simulated and real network data sets.Large-scale model selection in misspecified generalized linear models
Demirkaya, E., Feng, Y., Basu, P., & Lv, J. (n.d.).Publication year
2022Journal title
BiometrikaVolume
109Issue
1Page(s)
123-136AbstractModel selection is crucial both to high-dimensional learning and to inference for contemporary big data applications in pinpointing the best set of covariates among a sequence of candidate interpretable models. Most existing work implicitly assumes that the models are correctly specified or have fixed dimensionality, yet both model misspecification and high dimensionality are prevalent in practice. In this paper, we exploit the framework of model selection principles under the misspecified generalized linear models presented in Lv Liu (2014), and investigate the asymptotic expansion of the posterior model probability in the setting of high-dimensional misspecified models. With a natural choice of prior probabilities that encourages interpretability and incorporates the Kullback-Leibler divergence, we suggest using the high-dimensional generalized Bayesian information criterion with prior probability for large-scale model selection with misspecification. Our new information criterion characterizes the impacts of both model misspecification and high dimensionality on model selection. We further establish the consistency of covariance contrast matrix estimation and the model selection consistency of the new information criterion in ultrahigh dimensions under some mild regularity conditions. Our numerical studies demonstrate that the proposed method enjoys improved model selection consistency over its main competitors.Model Averaging for Nonlinear Regression Models
Feng, Y., Liu, Q., Yao, Q., & Zhao, G. (n.d.).Publication year
2022Journal title
Journal of Business and Economic StatisticsVolume
40Issue
2Page(s)
785-798AbstractThis article considers the problem of model averaging for regression models that can be nonlinear in their parameters and variables. We consider a nonlinear model averaging (NMA) framework and propose a weight-choosing criterion, the nonlinear information criterion (NIC). We show that up to a constant, NIC is an asymptotically unbiased estimator of the risk function under nonlinear settings with some mild assumptions. We also prove the optimality of NIC and show the convergence of the model averaging weights. Monte Carlo experiments reveal that NMA leads to relatively lower risks compared with alternative model selection and model averaging methods in most situations. Finally, we apply the NMA method to predicting the individual wage, where our approach leads to the lowest prediction errors in most cases.Targeting Predictors Via Partial Distance Correlation With Applications to Financial Forecasting
Yousuf, K., & Feng, Y. (n.d.).Publication year
2022Journal title
Journal of Business and Economic StatisticsVolume
40Issue
3Page(s)
1007-1019AbstractHigh-dimensional time series datasets are becoming increasingly common in various fields of economics and finance. Given the ubiquity of time series data, it is crucial to develop efficient variable screening methods that use the unique features of time series. This article introduces several model-free screening methods based on partial distance correlation and developed specifically to deal with time-dependent data. Methods are developed both for univariate models, such as nonlinear autoregressive models with exogenous predictors (NARX), and multivariate models such as linear or nonlinear VAR models. Sure screening properties are proved for our methods, which depend on the moment conditions, and the strength of dependence in the response and covariate processes, amongst other factors. We show the effectiveness of our methods via extensive simulation studies and an application on forecasting U.S. market returns.TESTING COMMUNITY STRUCTURE FOR HYPERGRAPHS
Yuan, M., Liu, R., Feng, Y., & Shang, Z. (n.d.).Publication year
2022Journal title
Annals of StatisticsVolume
50Issue
1Page(s)
147-169AbstractMany complex networks in the real world can be formulated as hypergraphs where community detection has been widely used. However, the fundamental question of whether communities exist or not in an observed hypergraph remains unclear. This work aims to tackle this important problem. Specifically, we systematically study when a hypergraph with community structure can be successfully distinguished from its Erdos-Rényi counterpart, and propose concrete test statistics when the models are distinguishable. The main contribution of this paper is threefold. First, we discover a phase transition in the hyperedge probability for distinguishability. Second, in the bounded-degree regime, we derive a sharp signal-to-noise ratio (SNR) threshold for distinguishability in the special two-community 3- uniform hypergraphs, and derive nearly tight SNR thresholds in the general two-community m-uniform hypergraphs. Third, in the dense regime, we propose a computationally feasible test based on sub-hypergraph counts, obtain its asymptotic distribution, and analyze its power. Our results are further extended to nonuniform hypergraphs in which a new test involving both edge and hyperedge information is proposed. The proofs rely on Janson's contiguity theory (Combin. Probab. Comput. 4 (1995) 369-405), a high-moments driven asymptotic normality result by Gao andWormald (Probab. Theory Related Fields 130 (2004) 368-376), and a truncation technique for analyzing the likelihood ratio.Analytical performance of lateral flow immunoassay for SARS-CoV-2 exposure screening on venous and capillary blood samples
Black, M. A., Shen, G., Feng, X., Garcia Beltran, W. F., Feng, Y., Vasudevaraja, V., Allison, D., Lin, L. H., Gindin, T., Astudillo, M., Yang, D., Murali, M., Iafrate, A. J., Jour, G., Cotzia, P., & Snuderl, M. (n.d.).Publication year
2021Journal title
Journal of Immunological MethodsVolume
489AbstractObjectives: We validate the use of a lateral flow immunoassay (LFI) intended for rapid screening and qualitative detection of anti-SARS-CoV-2 IgM and IgG in serum, plasma, and whole blood, and compare results with ELISA. We also seek to establish the value of LFI testing on blood obtained from a capillary blood sample. Methods: Samples collected by venous blood draw and finger stick were obtained from patients with SARS-CoV-2 detected by RT-qPCR and control patients. Samples were tested with Biolidics 2019-nCoV IgG/IgM Detection Kit lateral flow immunoassay, and antibody calls were compared with ELISA. Results: Biolidics LFI showed clinical sensitivity of 92% with venous blood at 7 days after PCR diagnosis of SARS-CoV-2. Test specificity was 92% for IgM and 100% for IgG. There was no significant difference in detecting IgM and IgG with Biolidics LFI and ELISA at D0 and D7 (p = 1.00), except for detection of IgM at D7 (p = 0.04). Capillary blood of SARS-CoV-2 patients showed 93% sensitivity for antibody detection. Conclusions: Clinical performance of Biolidics 2019-nCoV IgG/IgM Detection Kit is comparable to ELISA and was consistent across sample types. This provides an opportunity for decentralized rapid testing and may allow point-of-care and longitudinal self-testing for the presence of anti-SARS-CoV-2 antibodies.Association of body composition parameters measured on CT with risk of hospitalization in patients with Covid-19
Chandarana, H., Pisuchpen, N., Krieger, R., Dane, B., Mikheev, A., Feng, Y., Kambadakone, A., & Rusinek, H. (n.d.).Publication year
2021Journal title
European Journal of RadiologyVolume
145AbstractPurpose: To assess prognostic value of body composition parameters measured at CT to predict risk of hospitalization in patients with COVID-19 infection. Methods: 177 patients with SARS-CoV-2 infection and with abdominopelvic CT were included in this retrospective IRB approved two-institution study. Patients were stratified based on disease severity as outpatients (no hospital admission) and patients who were hospitalized (inpatients). Two readers blinded to the clinical outcome segmented axial CT images at the L3 vertebral body level for visceral adipose tissue (VAT), subcutaneous adipose tissue (SAT), muscle adipose tissue (MAT), muscle mass (MM). VAT to total adipose tissue ratio (VAT/TAT), MAT/MM ratio, and muscle index (MI) at L3 were computed. These measures, along with detailed clinical risk factors, were compared in patients stratified by severity. Various logistic regression clinical and clinical + imaging models were compared to discriminate inpatients from outpatients. Results: There were 76 outpatients (43%) and 101 inpatients. Male gender (p = 0.013), age (p = 0.0003), hypertension (p = 0.0003), diabetes (p = 0.0001), history of cardiac disease (p = 0.007), VAT/TAT (p < 0.0001), and MAT/MM (p < 0.0001), but not BMI, were associated with hospitalization. A clinical model (age, gender, BMI) had AUC of 0.70. Addition of VAT/TAT to the clinical model improved the AUC to 0.73. Optimal model that included gender, BMI, race (Black), MI, VAT/TAT, as well as interaction between gender and VAT/TAT and gender and MAT/MM demonstrated the highest AUC of 0.83. Conclusion: MAT/MM and VAT/TAT provides important prognostic information in predicting patients with COVID-19 who are likely to require hospitalization.Comparison of solid tissue sequencing and liquid biopsy accuracy in identification of clinically relevant gene mutations and rearrangements in lung adenocarcinomas
Lin, L. H., Allison, D. H., Feng, Y., Jour, G., Park, K., Zhou, F., Moreira, A. L., Shen, G., Feng, X., Sabari, J., Velcheti, V., Snuderl, M., & Cotzia, P. (n.d.).Publication year
2021Journal title
Modern PathologyVolume
34Issue
12Page(s)
2168-2174AbstractScreening for therapeutic targets is standard of care in the management of advanced non-small cell lung cancer. However, most molecular assays utilize tumor tissue, which may not always be available. “Liquid biopsies” are plasma-based next generation sequencing (NGS) assays that use circulating tumor DNA to identify relevant targets. To compare the sensitivity, specificity, and accuracy of a plasma-based NGS assay to solid-tumor-based NGS we retrospectively analyzed sequencing results of 100 sequential patients with lung adenocarcinoma at our institution who had received concurrent testing with both a solid-tissue-based NGS assay and a commercially available plasma-based NGS assay. Patients represented both new diagnoses (79%) and disease progression on treatment (21%); the majority (83%) had stage IV disease. Tissue-NGS identified 74 clinically relevant mutations, including 52 therapeutic targets, a sensitivity of 94.8%, while plasma-NGS identified 41 clinically relevant mutations, a sensitivity of 52.6% (p < 0.001). Tissue-NGS showed significantly higher sensitivity and accuracy across multiple patient subgroups, both in newly diagnosed and treated patients, as well as in metastatic and nonmetastatic disease. Discrepant cases involved hotspot mutations and actionable fusions including those in EGFR, ALK, and NTRK1. In summary, tissue-NGS detects significantly more clinically relevant alterations and therapeutic targets compared to plasma-NGS, suggesting that tissue-NGS should be the preferred method for molecular testing of lung adenocarcinoma when tissue is available. Plasma-NGS can still play an important role when tissue testing is not possible. However, given its low sensitivity, a negative result should be confirmed with a tissue-based assay.Imbalanced classification: A paradigm-based review
Feng, Y., Zhou, M., & Tong, X. (n.d.).Publication year
2021Journal title
Statistical Analysis and Data MiningVolume
14Issue
5Page(s)
383-406AbstractA common issue for classification in scientific research and industry is the existence of imbalanced classes. When sample sizes of different classes are imbalanced in training data, naively implementing a classification method often leads to unsatisfactory prediction results on test data. Multiple resampling techniques have been proposed to address the class imbalance issues. Yet, there is no general guidance on when to use each technique. In this article, we provide a paradigm-based review of the common resampling techniques for binary classification under imbalanced class sizes. The paradigms we consider include the classical paradigm that minimizes the overall classification error, the cost-sensitive learning paradigm that minimizes a cost-adjusted weighted type I and type II errors, and the Neyman–Pearson paradigm that minimizes the type II error subject to a type I error constraint. Under each paradigm, we investigate the combination of the resampling techniques and a few state-of-the-art classification methods. For each pair of resampling techniques and classification methods, we use simulation studies and a real dataset on credit card fraud to study the performance under different evaluation metrics. From these extensive numerical experiments, we demonstrate under each classification paradigm, the complex dynamics among resampling techniques, base classification methods, evaluation metrics, and imbalance ratios. We also summarize a few takeaway messages regarding the choices of resampling techniques and base classification methods, which could be helpful for practitioners.Mediation effect selection in high-dimensional and compositional microbiome data
Zhang, H., Chen, J., Feng, Y., Wang, C., Li, H., & Liu, L. (n.d.).Publication year
2021Journal title
Statistics in MedicineVolume
40Issue
4Page(s)
885-896AbstractThe microbiome plays an important role in human health by mediating the path from environmental exposures to health outcomes. The relative abundances of the high-dimensional microbiome data have an unit-sum restriction, rendering standard statistical methods in the Euclidean space invalid. To address this problem, we use the isometric log-ratio transformations of the relative abundances as the mediator variables. To select significant mediators, we consider a closed testing-based selection procedure with desirable confidence. Simulations are provided to verify the effectiveness of our method. As an illustrative example, we apply the proposed method to study the mediation effects of murine gut microbiome between subtherapeutic antibiotic treatment and body weight gain, and identify Coprobacillus and Adlercreutzia as two significant mediators.RaSE: Random subspace ensemble classification
Tian, Y., & Feng, Y. (n.d.).Publication year
2021Journal title
Journal of Machine Learning ResearchVolume
22AbstractWe propose a exible ensemble classification framework, Random Subspace Ensemble (RaSE), for sparse classification. In the RaSE algorithm, we aggregate many weak learners, where each weak learner is a base classifier trained in a subspace optimally selected from a collection of random subspaces. To conduct subspace selection, we propose a new criterion, ratio information criterion (RIC), based on weighted Kullback-Leibler divergence. The theoretical analysis includes the risk and Monte-Carlo variance of the RaSE classifier, establishing the screening consistency and weak consistency of RIC, and providing an upper bound for the misclassification rate of the RaSE classifier. In addition, we show that in a high-dimensional framework, the number of random subspaces needs to be very large to guarantee that a subspace covering signals is selected. Therefore, we propose an iterative version of the RaSE algorithm and prove that under some specific conditions, a smaller number of generated random subspaces are needed to find a desirable subspace through iteration. An array of simulations under various models and real-data applications demonstrate the effectiveness and robustness of the RaSE classifier and its iterative version in terms of low misclassification rate and accurate feature ranking. The RaSE algorithm is implemented in the R package RaSEn on CRAN.