Yang Feng
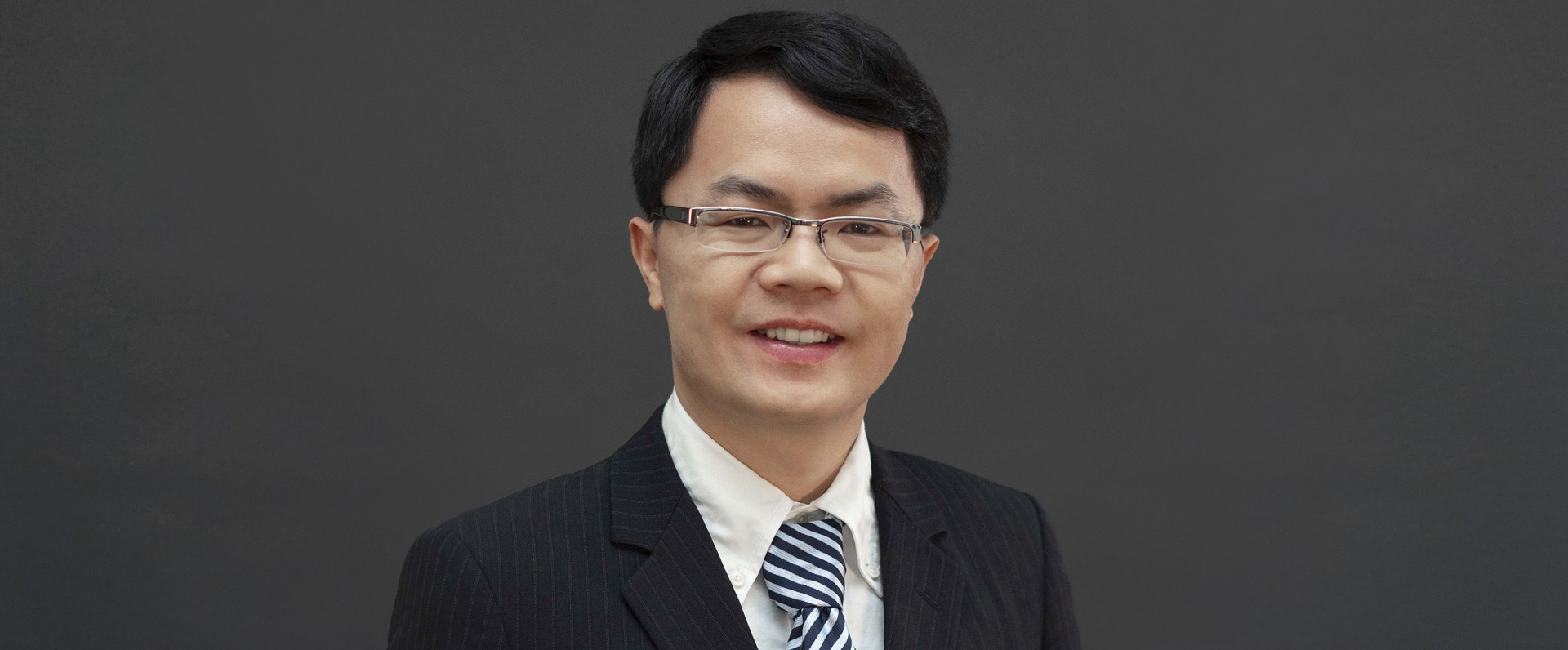
Yang Feng
Professor of Biostatistics
-
Professional overview
-
Yang Feng is a Professor and Ph.D. Program Director of Biostatistics in the School of Global Public Health and an affiliate faculty in the Center for Data Science at New York University. He obtained his Ph.D. in Operations Research at Princeton University in 2010.
Feng's research interests encompass the theoretical and methodological aspects of machine learning, high-dimensional statistics, social network models, and nonparametric statistics, leading to a wealth of practical applications, including Alzheimer's disease, cancer classification, and electronic health records. His research has been funded by multiple grants from the National Institutes of Health (NIH) and the National Science Foundation (NSF), notably the NSF CAREER Award.
He is currently an Associate Editor for the Journal of the American Statistical Association (JASA), the Journal of Business & Economic Statistics (JBES), Journal of Computational & Graphical Statistics (JCGS), and the Annals of Applied Statistics (AoAS). His professional recognitions include being named a fellow of the American Statistical Association (ASA) and the Institute of Mathematical Statistics (IMS), as well as an elected member of the International Statistical Institute (ISI).
Please visit Dr. Yang Feng's website and Google Scholar page from more information.
-
Education
-
B.S. in Mathematics, University of Science and Technology of China, Hefei, ChinaPh.D. in Operations Research, Princeton University, Princeton, NJ
-
Areas of research and study
-
BioinformaticsBiostatisticsHigh-dimensional data analysis/integrationMachine learningModeling Social and Behavioral DynamicsNonparametric statistics
-
Publications
Publications
JDINAC: Joint density-based non-parametric differential interaction network analysis and classification using high-dimensional sparse omics data
Ji, J., He, D., Feng, Y., He, Y., Xue, F., & Xie, L. (n.d.).Publication year
2017Journal title
BioinformaticsVolume
33Issue
19Page(s)
3080-3087AbstractMotivation A complex disease is usually driven by a number of genes interwoven into networks, rather than a single gene product. Network comparison or differential network analysis has become an important means of revealing the underlying mechanism of pathogenesis and identifying clinical biomarkers for disease classification. Most studies, however, are limited to network correlations that mainly capture the linear relationship among genes, or rely on the assumption of a parametric probability distribution of gene measurements. They are restrictive in real application. Results We propose a new Joint density based non-parametric Differential Interaction Network Analysis and Classification (JDINAC) method to identify differential interaction patterns of network activation between two groups. At the same time, JDINAC uses the network biomarkers to build a classification model. The novelty of JDINAC lies in its potential to capture non-linear relations between molecular interactions using high-dimensional sparse data as well as to adjust confounding factors, without the need of the assumption of a parametric probability distribution of gene measurements. Simulation studies demonstrate that JDINAC provides more accurate differential network estimation and lower classification error than that achieved by other state-of-the-art methods. We apply JDINAC to a Breast Invasive Carcinoma dataset, which includes 114 patients who have both tumor and matched normal samples. The hub genes and differential interaction patterns identified were consistent with existing experimental studies. Furthermore, JDINAC discriminated the tumor and normal sample with high accuracy by virtue of the identified biomarkers. JDINAC provides a general framework for feature selection and classification using high-dimensional sparse omics data.Post selection shrinkage estimation for high-dimensional data analysis
Gao, X., Ahmed, S. E., & Feng, Y. (n.d.).Publication year
2017Journal title
Applied Stochastic Models in Business and IndustryVolume
33Issue
2Page(s)
97-120AbstractIn high-dimensional data settings where p ≫ n, many penalized regularization approaches were studied for simultaneous variable selection and estimation. However, with the existence of covariates with weak effect, many existing variable selection methods, including Lasso and its generations, cannot distinguish covariates with weak and no contribution. Thus, prediction based on a subset model of selected covariates only can be inefficient. In this paper, we propose a post selection shrinkage estimation strategy to improve the prediction performance of a selected subset model. Such a post selection shrinkage estimator (PSE) is data adaptive and constructed by shrinking a post selection weighted ridge estimator in the direction of a selected candidate subset. Under an asymptotic distributional quadratic risk criterion, its prediction performance is explored analytically. We show that the proposed post selection PSE performs better than the post selection weighted ridge estimator. More importantly, it improves the prediction performance of any candidate subset model selected from most existing Lasso-type variable selection methods significantly. The relative performance of the post selection PSE is demonstrated by both simulation studies and real-data analysis.Rejoinder to ‘Post-selection shrinkage estimation for high-dimensional data analysis’
Gao, X., Ejaz Ahmed, S., & Feng, Y. (n.d.).Publication year
2017Journal title
Applied Stochastic Models in Business and IndustryVolume
33Issue
2Page(s)
131-135AbstractRejoinder to the paper entitled ‘Post-selection shrinkage estimation for high-dimensional data analysis’ discusses different aspects of the study. One fundamental ingredient of the work is to formally split the signals into strong and weak ones. The rationale is that the usual one-step method such as the least absolute shrinkage and selection operator (LASSO) may be very effective in detecting strong signals while failing to identify some weak ones, which in turn has a significant impact on the model fitting, as well as prediction. The discussions of both Fan and QYY contain very interesting comments on the separation of the three sets of variables.A survey on Neyman-Pearson classification and suggestions for future research
Tong, X., Feng, Y., & Zhao, A. (n.d.).Publication year
2016Journal title
Wiley Interdisciplinary Reviews: Computational StatisticsVolume
8Issue
2Page(s)
64-81AbstractIn statistics and machine learning, classification studies how to automatically learn to make good qualitative predictions (i.e., assign class labels) based on past observations. Examples of classification problems include email spam filtering, fraud detection, market segmentation. Binary classification, in which the potential class label is binary, has arguably the most widely used machine learning applications. Most existing binary classification methods target on the minimization of the overall classification risk and may fail to serve some real-world applications such as cancer diagnosis, where users are more concerned with the risk of misclassifying one specific class than the other. Neyman-Pearson (NP) paradigm was introduced in this context as a novel statistical framework for handling asymmetric type I/II error priorities. It seeks classifiers with a minimal type II error subject to a type I error constraint under some user-specified level. Though NP classification has the potential to be an important subfield in the classification literature, it has not received much attention in the statistics and machine learning communities. This article is a survey on the current status of the NP classification literature. To stimulate readers’ research interests, the authors also envision a few possible directions for future research in NP paradigm and its applications.Feature Augmentation via Nonparametrics and Selection (FANS) in High-Dimensional Classification
Fan, J., Feng, Y., Jiang, J., & Tong, X. (n.d.).Publication year
2016Journal title
Journal of the American Statistical AssociationVolume
111Issue
513Page(s)
275-287AbstractWe propose a high-dimensional classification method that involves nonparametric feature augmentation. Knowing that marginal density ratios are the most powerful univariate classifiers, we use the ratio estimates to transform the original feature measurements. Subsequently, penalized logistic regression is invoked, taking as input the newly transformed or augmented features. This procedure trains models equipped with local complexity and global simplicity, thereby avoiding the curse of dimensionality while creating a flexible nonlinear decision boundary. The resulting method is called feature augmentation via nonparametrics and selection (FANS). We motivate FANS by generalizing the naive Bayes model, writing the log ratio of joint densities as a linear combination of those of marginal densities. It is related to generalized additive models, but has better interpretability and computability. Risk bounds are developed for FANS. In numerical analysis, FANS is compared with competing methods, so as to provide a guideline on its best application domain. Real data analysis demonstrates that FANS performs very competitively on benchmark email spam and gene expression datasets. Moreover, FANS is implemented by an extremely fast algorithm through parallel computing.Neyman-pearson classiffication under high-dimensional settings
Zhao, A., Feng, Y., Wang, L., & Tong, X. (n.d.).Publication year
2016Journal title
Journal of Machine Learning ResearchVolume
17Page(s)
1-39AbstractMost existing binary classiffication methods target on the optimization of the overall classification risk and may fail to serve some real-world applications such as cancer diagnosis, where users are more concerned with the risk of misclassifying one speciffic class than the other. Neyman-Pearson (NP) paradigm was introduced in this context as a novel statistical framework for handling asymmetric type I/II error priorities. It seeks classifiers with a minimal type II error and a constrained type I error under a user specified level. This article is the first attempt to construct classifiers with guaranteed theoretical performance under the NP paradigm in high-dimensional settings. Based on the fundamental Neyman-Pearson Lemma, we used a plug-in approach to construct NP-Type classifiers for Naive Bayes models. The proposed classifiers satisfy the NP oracle inequalities, which are natural NP paradigm counterparts of the oracle inequalities in classical binary classification. Besides their desirable theoretical properties, we also demonstrated their numerical advantages in prioritized error control via both simulation and real data studies.Tuning-parameter selection in regularized estimations of large covariance matrices
Fang, Y., Wang, B., & Feng, Y. (n.d.).Publication year
2016Journal title
Journal of Statistical Computation and SimulationVolume
86Issue
3Page(s)
494-509AbstractRecently many regularized estimators of large covariance matrices have been proposed, and the tuning parameters in these estimators are usually selected via cross-validation. However, there is a lack of consensus on the number of folds for conducting cross-validation. One round of cross-validation involves partitioning a sample of data into two complementary subsets, a training set and a validation set. In this manuscript, we demonstrate that if the estimation accuracy is measured in the Frobenius norm, the training set should consist of majority of the data; whereas if the estimation accuracy is measured in the operator norm, the validation set should consist of majority of the data. We also develop methods for selecting tuning parameters based on the bootstrap and compare them with their cross-validation counterparts. We demonstrate that the cross-validation methods with ‘optimal’ choices of folds are more appropriate than their bootstrap counterparts.Variable selection and prediction with incomplete high-dimensional data
Liu, Y., Wang, Y., Feng, Y., & Wall, M. M. (n.d.).Publication year
2016Journal title
Annals of Applied StatisticsVolume
10Issue
1Page(s)
418-450AbstractWe propose a Multiple Imputation Random Lasso (MIRL) method to select important variables and to predict the outcome for an epidemiological study of Eating and Activity in Teens. In this study 80% of individuals have at least one variable missing. Therefore, using variable selection methods developed for complete data after listwise deletion substantially reduces prediction power. Recent work on prediction models in the presence of incomplete data cannot adequately account for large numbers of variables with arbitrary missing patterns. We propose MIRL to combine penalized regression techniques with multiple imputation and stability selection. Extensive simulation studies are conducted to compare MIRL with several alternatives. MIRL outperforms other methods in high-dimensional scenarios in terms of both reduced prediction error and improved variable selection performance, and it has greater advantage when the correlation among variables is high and missing proportion is high. MIRL is shown to have improved performance when comparing with other applicable methods when applied to the study of Eating and Activity in Teens for the boys and girls separately, and to a subgroup of low social economic status (SES) Asian boys who are at high risk of developing obesity.Functional and Parametric Estimation in a Semi-and Nonparametric Model with Application to Mass-Spectrometry Data
Ma, W., Feng, Y., Chen, K., & Ying, Z. (n.d.).Publication year
2015Journal title
International Journal of BiostatisticsVolume
11Issue
2Page(s)
285-303AbstractMotivated by modeling and analysis of mass-spectrometry data, a semi-and nonparametric model is proposed that consists of linear parametric components for individual location and scale and a nonparametric regression function for the common shape. A multi-step approach is developed that simultaneously estimates the parametric components and the nonparametric function. Under certain regularity conditions, it is shown that the resulting estimators is consistent and asymptotic normal for the parametric part and achieve the optimal rate of convergence for the nonparametric part when the bandwidth is suitably chosen. Simulation results are presented to demonstrate the effectiveness and finite-sample performance of the method. The method is also applied to a SELDI-TOF mass spectrometry data set from a study of liver cancer patients.APPLE: Approximate path for penalized likelihood estimators
Yu, Y., & Feng, Y. (n.d.).Publication year
2014Journal title
Statistics and ComputingVolume
24Issue
5Page(s)
803-819AbstractIn high-dimensional data analysis, penalized likelihood estimators are shown to provide superior results in both variable selection and parameter estimation. A new algorithm, APPLE, is proposed for calculating the Approximate Path for Penalized Likelihood Estimators. Both convex penalties (such as LASSO) and folded concave penalties (such as MCP) are considered. APPLE efficiently computes the solution path for the penalized likelihood estimator using a hybrid of the modified predictor-corrector method and the coordinate-descent algorithm. APPLE is compared with several well-known packages via simulation and analysis of two gene expression data sets.Modified Cross-Validation for Penalized High-Dimensional Linear Regression Models
Yu, Y., & Feng, Y. (n.d.).Publication year
2014Journal title
Journal of Computational and Graphical StatisticsVolume
23Issue
4Page(s)
1009-1027AbstractIn this article, for Lasso penalized linear regression models in high-dimensional settings, we propose a modified cross-validation (CV) method for selecting the penalty parameter. The methodology is extended to other penalties, such as Elastic Net. We conduct extensive simulation studies and real data analysis to compare the performance of the modified CV method with other methods. It is shown that the popular K-fold CV method includes many noise variables in the selected model, while the modified CV works well in a wide range of coefficient and correlation settings. Supplementary materials containing the computer code are available online.Regularized principal components of heritability
Fang, Y., Feng, Y., & Yuan, M. (n.d.).Publication year
2014Journal title
Computational StatisticsVolume
29Issue
3Page(s)
455-465AbstractIn family studies with multiple continuous phenotypes, heritability can be conveniently evaluated through the so-called principal-component of heredity (PCH, for short; Ott and Rabinowitz in Hum Hered 49:106-111, 1999). Estimation of the PCH, however, is notoriously difficult when entertaining a large collection of phenotypes which naturally arises in dealing with modern genomic data such as those from expression QTL studies. In this paper, we propose a regularized PCH method to specifically address such challenges. We show through both theoretical studies and data examples that the proposed method can accurately assess the heritability of a large collection of phenotypes.A road to classification in high dimensional space: The regularized optimal affine discriminant
Fan, J., Feng, Y., & Tong, X. (n.d.).Publication year
2012Journal title
Journal of the Royal Statistical Society. Series B: Statistical MethodologyVolume
74Issue
4Page(s)
745-771AbstractFor high dimensional classification, it is well known that naively performing the Fisher discriminant rule leads to poor results due to diverging spectra and accumulation of noise. Therefore, researchers proposed independence rules to circumvent the diverging spectra, and sparse independence rules to mitigate the issue of accumulation of noise. However, in biological applications, often a group of correlated genes are responsible for clinical outcomes, and the use of the covariance information can significantly reduce misclassification rates. In theory the extent of such error rate reductions is unveiled by comparing the misclassification rates of the Fisher discriminant rule and the independence rule. To materialize the gain on the basis of finite samples, a regularized optimal affine discriminant (ROAD) is proposed. The ROAD selects an increasing number of features as the regularization relaxes. Further benefits can be achieved when a screening method is employed to narrow the feature pool before applying the ROAD method. An efficient constrained co-ordinate descent algorithm is also developed to solve the associated optimization problems. Sampling properties of oracle type are established. Simulation studies and real data analysis support our theoretical results and demonstrate the advantages of the new classification procedure under a variety of correlation structures. A delicate result on continuous piecewise linear solution paths for the ROAD optimization problem at the population level justifies the linear interpolation of the constrained co-ordinate descent algorithm.Nonparametric independence screening in sparse ultra-high-dimensional additive models
Fan, J., Feng, Y., & Song, R. (n.d.).Publication year
2011Journal title
Journal of the American Statistical AssociationVolume
106Issue
494Page(s)
544-557AbstractA variable screening procedure via correlation learning was proposed by Fan and Lv (2008) to reduce dimensionality in sparse ultra-highdimensional models. Even when the true model is linear, the marginal regression can be highly nonlinear. To address this issue, we further extend the correlation learning to marginal nonparametric learning. Our nonparametric independence screening (NIS) is a specific type of sure independence screening. We propose several closely related variable screening procedures. We show that with general nonparametric models, under some mild technical conditions, the proposed independence screening methods have a sure screening property. The extentto which the dimensionality can be reduced by independence screening is also explicitly quantified. As a methodological extension, we also propose a data-driven thresholding and an iterative nonparametric independence screening (INIS) method to enhance the finite- sample performance for fitting sparse additive models. The simulation results and a real data analysis demonstrate that the proposed procedure works well with moderate sample size and large dimension and performs better than competing methods.Nonparametric estimation of genewise variance for microarray data
Fan, J., Feng, Y., & Niu, Y. S. (n.d.).Publication year
2010Journal title
Annals of StatisticsVolume
38Issue
5Page(s)
2723-2750AbstractEstimation of genewise variance arises from two important applications in microarray data analysis: selecting significantly differentially expressed genes and validation tests for normalization of microarray data. We approach the problem by introducing a two-way nonparametric model, which is an extension of the famous Neyman-Scott model and is applicable beyond microarray data. The problem itself poses interesting challenges because thenumber of nuisance parameters is proportional to the sample size and it is not obvious how the variance function can be estimated when measurements are correlated. In such a high-dimensional nonparametric problem, we proposed two novel nonparametric estimators for genewise variance function and semiparametric estimators for measurement correlation, via solving a system of nonlinear equations. Their asymptotic normality is established. The finite sample property is demonstrated by simulation studies. The estimators also improve the power of the tests for detecting statistically differentially expressed genes. The methodology is illustrated by the data from microarray quality control (MAQC) project.The Microarray Quality Control (MAQC)-II study of common practices for the development and validation of microarray-based predictive models
Shi, L., Campbell, G., Jones, W. D., Campagne, F., Wen, Z., Walker, S. J., Su, Z., Chu, T. M., Goodsaid, F. M., Pusztai, L., Shaughnessy, J. D., Oberthuer, A., Thomas, R. S., Paules, R. S., Fielden, M., Barlogie, B., Chen, W., Du, P., Fischer, M., … Wolfinger, R. D. (n.d.).Publication year
2010Journal title
Nature BiotechnologyVolume
28Issue
8Page(s)
827-838AbstractGene expression data from microarrays are being applied to predict preclinical and clinical endpoints, but the reliability of these predictions has not been established. In the MAQC-II project, 36 independent teams analyzed six microarray data sets to generate predictive models for classifying a sample with respect to one of 13 endpoints indicative of lung or liver toxicity in rodents, or of breast cancer, multiple myeloma or neuroblastoma in humans. In total, >30,000 models were built using many combinations of analytical methods. The teams generated predictive models without knowing the biological meaning of some of the endpoints and, to mimic clinical reality, tested the models on data that had not been used for training. We found that model performance depended largely on the endpoint and team proficiency and that different approaches generated models of similar performance. The conclusions and recommendations from MAQC-II should be useful for regulatory agencies, study committees and independent investigators that evaluate methods for global gene expression analysis.Local quasi-likelihood with a parametric guide
Fan, J., Wu, Y., & Feng, Y. (n.d.).Publication year
2009Journal title
Annals of StatisticsVolume
37Issue
6Page(s)
4153-4183AbstractGeneralized linear models and the quasi-likelihood method extend the ordinary regression models to accommodate more general conditional distributions of the response. Nonparametric methods need no explicit parametric specification, and the resulting model is completely determined by the data themselves. However, nonparametric estimation schemes generally have a slower convergence rate such as the local polynomial smoothing estimation of nonparametric generalized linear models studied in Fan, Heckman and Wand [J. Amer. Statist. Assoc. 90.Network exploration via the adaptive LASSO and SCAD penalties
Fan, J., Feng, Y., & Wu, Y. (n.d.).Publication year
2009Journal title
Annals of Applied StatisticsVolume
3Issue
2Page(s)
521-541AbstractGraphical models are frequently used to explore networks, such as genetic networks, among a set of variables. This is usually carried out via exploring the sparsity of the precision matrix of the variables under consideration. Penalized likelihood methods are often used in such explorations. Yet, positive-definiteness constraints of precision matrices make the optimization problem challenging. We introduce nonconcave penalties and the adaptive LASSO penalty to attenuate the bias problem in the network estimation. Through the local linear approximation to the nonconcave penalty functions, the problem of precision matrix estimation is recast as a sequence of penalized likelihood problems with a weighted L 1 penalty and solved using the efficient algorithm of Friedman et al. [Biostatistics 9 (2008) 432-441]. Our estimation schemes are applied to two real datasets. Simulation experiments and asymptotic theory are used to justify our proposed methods.