Yang Feng
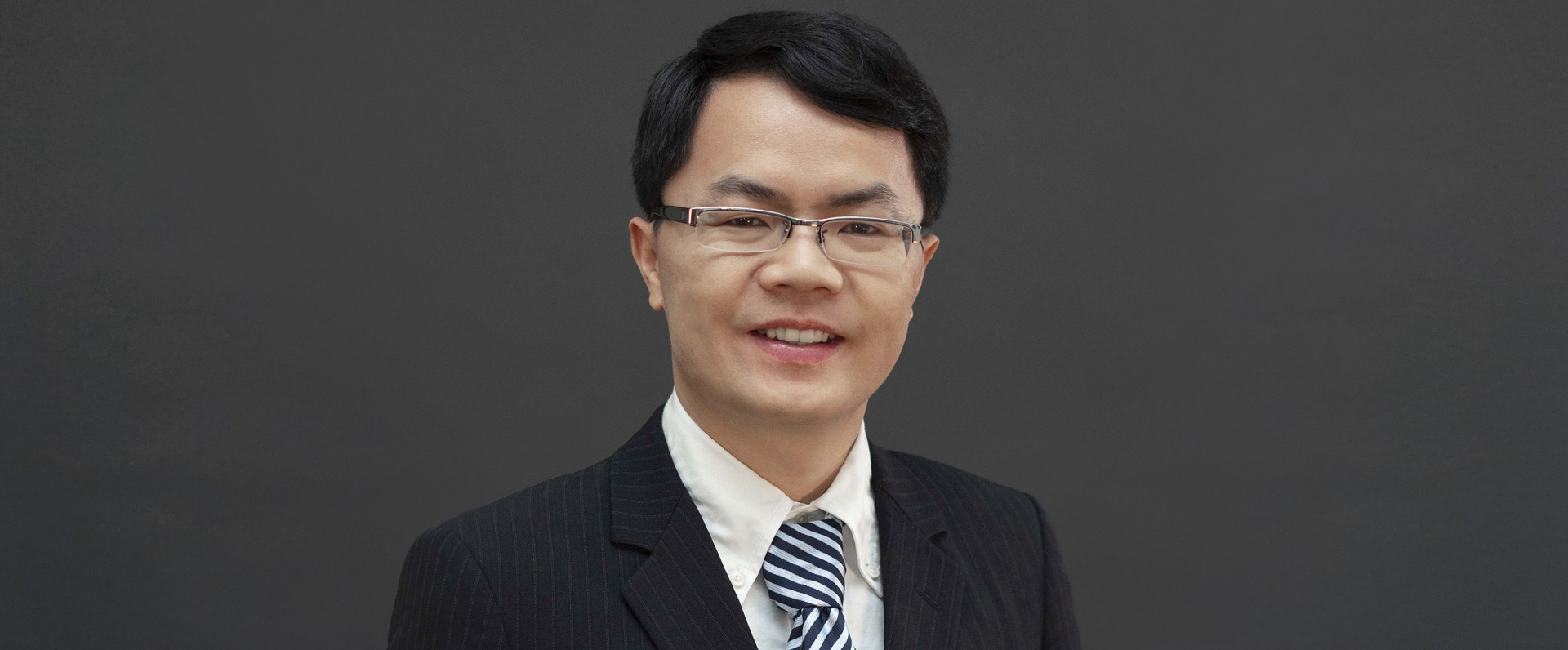
Yang Feng
Professor of Biostatistics
-
Professional overview
-
Yang Feng is a Professor and Ph.D. Program Director of Biostatistics in the School of Global Public Health and an affiliate faculty in the Center for Data Science at New York University. He obtained his Ph.D. in Operations Research at Princeton University in 2010.
Feng's research interests encompass the theoretical and methodological aspects of machine learning, high-dimensional statistics, social network models, and nonparametric statistics, leading to a wealth of practical applications, including Alzheimer's disease, cancer classification, and electronic health records. His research has been funded by multiple grants from the National Institutes of Health (NIH) and the National Science Foundation (NSF), notably the NSF CAREER Award.
He is currently an Associate Editor for the Journal of the American Statistical Association (JASA), the Journal of Business & Economic Statistics (JBES), Journal of Computational & Graphical Statistics (JCGS), and the Annals of Applied Statistics (AoAS). His professional recognitions include being named a fellow of the American Statistical Association (ASA) and the Institute of Mathematical Statistics (IMS), as well as an elected member of the International Statistical Institute (ISI).
Please visit Dr. Yang Feng's website and Google Scholar page from more information.
-
Education
-
B.S. in Mathematics, University of Science and Technology of China, Hefei, ChinaPh.D. in Operations Research, Princeton University, Princeton, NJ
-
Areas of research and study
-
BioinformaticsBiostatisticsHigh-dimensional data analysis/integrationMachine learningModeling Social and Behavioral DynamicsNonparametric statistics
-
Publications
Publications
Comparison of solid tissue sequencing and liquid biopsy accuracy in identification of clinically relevant gene mutations and rearrangements in lung adenocarcinomas
Imbalanced classification: A paradigm-based review
Mediation effect selection in high-dimensional and compositional microbiome data
RaSE: Random subspace ensemble classification
Super RaSE: Super Random Subspace Ensemble Classification
The Interplay of Demographic Variables and Social Distancing Scores in Deep Prediction of U.S. COVID-19 Cases
Visceral adipose tissue in patients with COVID-19: risk stratification for severity
A projection-based conditional dependence measure with applications to high-dimensional undirected graphical models
Fan, J., Feng, Y., & Xia, L. (n.d.).Publication year
2020Journal title
Journal of EconometricsVolume
218Issue
1Page(s)
119-139AbstractMeasuring conditional dependence is an important topic in econometrics with broad applications including graphical models. Under a factor model setting, a new conditional dependence measure based on projection is proposed. The corresponding conditional independence test is developed with the asymptotic null distribution unveiled where the number of factors could be high-dimensional. It is also shown that the new test has control over the asymptotic type I error and can be calculated efficiently. A generic method for building dependency graphs without Gaussian assumption using the new test is elaborated. We show the superiority of the new method, implemented in the R package pgraph, through simulation and real data studies.Accounting for incomplete testing in the estimation of epidemic parameters
Nested model averaging on solution path for high-dimensional linear regression
Feng, Y., & Liu, Q. (n.d.).Publication year
2020Journal title
StatVolume
9Issue
1AbstractWe study the nested model averaging method on the solution path for a high-dimensional linear regression problem. In particular, we propose to combine model averaging with regularized estimators (e.g., lasso, elastic net, and Sorted L-One Penalized Estimation [SLOPE]) on the solution path for high-dimensional linear regression. In simulation studies, we first conduct a systematic investigation on the impact of predictor ordering on the behaviour of nested model averaging, and then show that nested model averaging with lasso, elastic net and SLOPE compares favourably with other competing methods, including the infeasible lasso, elastic, net and SLOPE with the tuning parameter optimally selected. A real data analysis on predicting the per capita violent crime in the United States shows outstanding performance of the nested model averaging with lasso.Neyman-pearson classification: Parametrics and sample size requirement
On the estimation of correlation in a binary sequence model
On the sparsity of Mallows model averaging estimator
A Kronecker Product Model for Repeated Pattern Detection on 2D Urban Images
Likelihood adaptively modified penalties
Feng, Y., Li, T., & Ying, Z. (n.d.).Publication year
2019Journal title
Applied Stochastic Models in Business and IndustryVolume
35Issue
2Page(s)
330-353AbstractA new family of penalty functions, ie, adaptive to likelihood, is introduced for model selection in general regression models. It arises naturally through assuming certain types of prior distribution on the regression parameters. To study the stability properties of the penalized maximum-likelihood estimator, 2 types of asymptotic stability are defined. Theoretical properties, including the parameter estimation consistency, model selection consistency, and asymptotic stability, are established under suitable regularity conditions. An efficient coordinate-descent algorithm is proposed. Simulation results and real data analysis show that the proposed approach has competitive performance in comparison with the existing methods.Regularization after retention in ultrahigh dimensional linear regression models
The restricted consistency property of leave-nV-out cross-validation for high-dimensional variable selection
A crowdsourced analysis to identify ab initio molecular signatures predictive of susceptibility to viral infection
Model Selection for High-Dimensional Quadratic Regression via Regularization
Neyman-Pearson classification algorithms and NP receiver operating characteristics
Nonparametric independence screening via favored smoothing bandwidth
Penalized weighted least absolute deviation regression
SIS: An R package for sure independence screening in ultrahigh-dimensional statistical models
Binary switch portfolio
Li, T., Chen, K., Feng, Y., & Ying, Z. (n.d.).Publication year
2017Journal title
Quantitative FinanceVolume
17Issue
5Page(s)
763-780AbstractWe propose herein a new portfolio selection method that switches between two distinct asset allocation strategies. An important component is a carefully designed adaptive switching rule, which is based on a machine learning algorithm. It is shown that using this adaptive switching strategy, the combined wealth of the new approach is a weighted average of that of the successive constant rebalanced portfolio and that of the 1/N portfolio. In particular, it is asymptotically superior to the 1/N portfolio under mild conditions in the long run. Applications to real data show that both the returns and the Sharpe ratios of the proposed binary switch portfolio are the best among several popular competing methods over varying time horizons and stock pools.How Many Communities Are There?