Shu Xu
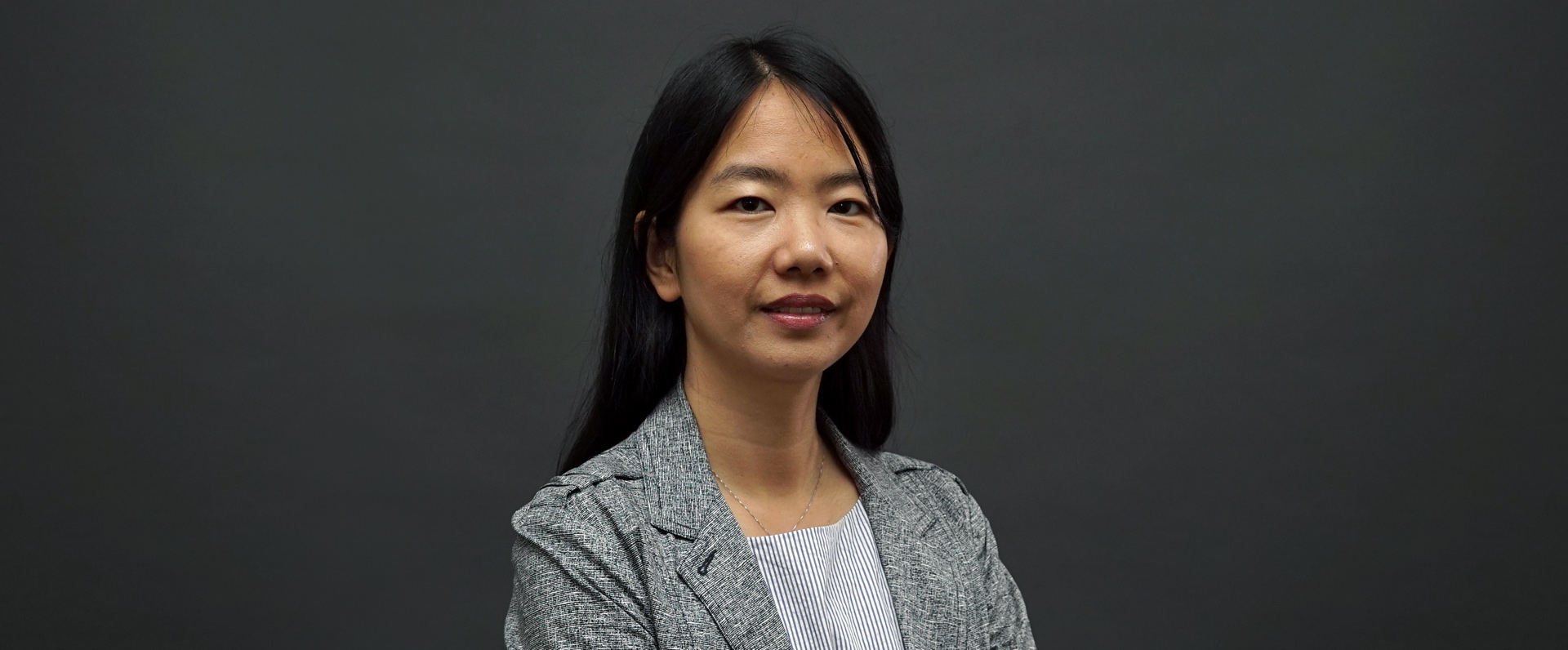
Shu Xu
Scroll
Clinical Associate Professor of Biostatistics
-
Professional overview
-
Dr. Shu Xu’s work represents a balance of both statistical and applied aspects of quantitative methodology. Her primary quantitative interests include evaluating and developing statistical methods for longitudinal data analysis. Specifically, Dr. Xu’s research focuses on various aspects of latent growth models, missing data methods, and causal inference models.
Dr. Xu has collaborated with substance use, family, and health researchers to advance and share her knowledge of quantitative methodology and pursue a better understanding of the social sciences and public health. She has conducted research with the Family Translational Research Group at NYU and the Methodology Center at the Pennsylvania State University.
-
Education
-
BS, Psychology, East China Normal University, Shanghai, ChinaMS, Quantitative Psychology, University of California, DavisPhD, Quantitative Psychology, University of California, Davis
-
Areas of research and study
-
BiostatisticsFamily researchLongitudinal Data AnalysisMissing Data MethodsMixture ModelsQuantitative Research
-
Publications
Publications
Noxious family environments in relation to adult and childhood caries
Lorber, M. F., Slep, A. M., Heyman, R. E., Xu, S., Dasanayake, A. P., & Wolff, M. S. (n.d.).Publication year
2014Journal title
Journal of the American Dental AssociationVolume
145Issue
9Page(s)
924-930AbstractBackground. The authors tested hypotheses that more noxious family environments are associated with poorer adult and child oral health. Methods. A community sample of married or cohabiting couples (N = 135) and their elementary school-aged children participated. Dental hygienists determined the number of decayed, missing and filled surfaces via oral examination. Subjective oral health impacts were measured by means of questionnaires completed by the parents and children. The parents completed questionnaires about interparental and parent-to-child physical aggression (for example, pushing) and emotional aggression (for example, derision), as well as harsh discipline. Observers rated the couples' hostile behavior in laboratory interactions. Results. The extent of women's and men's caries experience was associated positively with their partners' levels of overall noxious behavior toward them. The extent of children's caries experience was associated positively with the level of their mothers' emotional aggression toward their partners. Conclusions. Noxious family environments may be implicated in compromised oral health. Future research that replicates and extends these findings can provide the foundation to translate them into preventive interventions. Practical Implications. Noxious family environments may help explain the limitations of routine oral health preventive strategies. Interprofessional strategies that also address the family environment ultimately may prove to be more effective than are single modality approaches.On Fitting a Multivariate Two-Part Latent Growth Model
Xu, S., Blozis, S. A., & Vandewater, E. A. (n.d.).Publication year
2014Journal title
Structural Equation ModelingVolume
21Issue
1Page(s)
131-148AbstractA 2-part latent growth model can be used to analyze semicontinuous data to simultaneously study change in the probability that an individual engages in a behavior, and if engaged, change in the behavior. This article uses a Monte Carlo (MC) integration algorithm to study the interrelationships between the growth factors of 2 variables measured longitudinally where each variable can follow a 2-part latent growth model. A SAS macro implementing Mplus is developed to estimate the model to take into account the sampling uncertainty of this simulation-based computational approach. A sample of time-use data is used to show how maximum likelihood estimates can be obtained using a rectangular numerical integration method and an MC integration method.Causal Inference in Latent Class Analysis
Lanza, S. T., Coffman, D. L., & Xu, S. (n.d.).Publication year
2013Journal title
Structural Equation ModelingVolume
20Issue
3Page(s)
361-383AbstractThe integration of modern methods for causal inference with latent class analysis (LCA) allows social, behavioral, and health researchers to address important questions about the determinants of latent class membership. In this article, 2 propensity score techniques, matching and inverse propensity weighting, are demonstrated for conducting causal inference in LCA. The different causal questions that can be addressed with these techniques are carefully delineated. An empirical analysis based on data from the National Longitudinal Survey of Youth 1979 is presented, where college enrollment is examined as the exposure (i.e., treatment) variable and its causal effect on adult substance use latent class membership is estimated. A step-by-step procedure for conducting causal inference in LCA, including multiple imputation of missing data on the confounders, exposure variable, and multivariate outcome, is included. Sample syntax for carrying out the analysis using SAS and R is given in an appendix.Preadolescent drug use resistance skill profiles, substance use, and substance use prevention
Hopfer, S., Hecht, M. L., Lanza, S. T., Tan, X., & Xu, S. (n.d.).Publication year
2013Journal title
Journal of Primary PreventionVolume
34Issue
6Page(s)
395-404AbstractThe aims of the current study were threefold: (1) specify the skills component of social influence prevention interventions for preadolescents, (2) examine the relationship between resistance skill profiles and substance use among preadolescents, and (3) evaluate whether subgroups of preadolescents based on their resistance skills and refusal confidence may be differentially impacted by the kiR prevention program. Latent class analysis showed a four-class model of 5th grader resistance skill profiles. Approximately half of preadolescents (53 %) were familiar with four prototypical resistance skills and showed confidence to apply these skills in real-world settings (highly competent profile); 15 % were familiar with resistance skills but had little confidence (skillful profile); 18 % were confident yet had little knowledge (confident profile); while 15 % had low knowledge and confidence (low competence profile). These skill profiles significantly predicted 8th grade recent substance use (2LL = -2,262.21, df = 3, p = .0005). As predicted by theory, the highly competent skill profile reported lower mean recent substance use than the population sample mean use. Latent transition analysis showed that although patterns of transiting into the highly competent skill profile over time were observed in the expected direction, this pattern was not significant when comparing treatment and control. Identifying skill profiles that predict recent substance use is theoretically consistent and has important implications for healthy and substance-free development.Sensitivity Analysis of Multiple Informant Models When Data Are Not Missing at Random
Blozis, S. A., Ge, X., Xu, S., Natsuaki, M. N., Shaw, D. S., Neiderhiser, J. M., Scaramella, L. V., Leve, L. D., & Reiss, D. (n.d.).Publication year
2013Journal title
Structural Equation ModelingVolume
20Issue
2Page(s)
283-298AbstractMissing data are common in studies that rely on multiple informant data to evaluate relationships among variables for distinguishable individuals clustered within groups. Estimation of structural equation models using raw data allows for incomplete data, and so all groups can be retained for analysis even if only 1 member of a group contributes data. Statistical inference is based on the assumption that data are missing completely at random or missing at random. Importantly, whether or not data are missing is assumed to be independent of the missing data. A saturated correlates model that incorporates correlates of the missingness or the missing data into an analysis and multiple imputation that might also use such correlates offer advantages over the standard implementation of SEM when data are not missing at random because these approaches could result in a data analysis problem for which the missingness is ignorable. This article considers these approaches in an analysis of family data to assess the sensitivity of parameter estimates and statistical inferences to assumptions about missing data, a strategy that could be easily implemented using SEM software.Sensitivity analysis of mixed models for incomplete longitudinal data
Xu, S., & Blozis, S. A. (n.d.).Publication year
2011Journal title
Journal of Educational and Behavioral StatisticsVolume
36Issue
2Page(s)
237-256AbstractMixed models are used for the analysis of data measured over time to study population-level change and individual differences in change characteristics. Linear and nonlinear functions may be used to describe a longitudinal response, individuals need not be observed at the same time points, and missing data, assumed to be missing at random (MAR), may be handled. While the mechanism giving rise to the missing data cannot be determined by the observations, the sensitivity of parameter estimates to missing data assumptions can be studied, for example, by fitting multiple models that make different assumptions about the missing data process. Sensitivity analysis of a mixed model that may include nonlinear parameters when some data are missing is discussed. An example is provided.Latent curve models: A structural equation perspective
Blozis, S. A., Cho, Y., & Xu, V. S. (n.d.).Publication year
2010Journal title
Sociological Methods and ResearchVolume
39Page(s)
297The belief and modeling of aging
Cui, L. J., Xu, V. S., & Wang, X. J. (n.d.).Publication year
2000Journal title
Chinese Journal of GerontologyVolume
20Page(s)
3老年人的养老观念与养老模式
Xu, V. S. (n.d.).Publication year
2000Journal title
中国老年学杂志Abstract目的 探讨老年人的养老观念与较理想的养老模式.方法 采用自编调查表对上海市居家养老老人,养老院或老年公寓养老老人以及老年人的子女进行了调查.结果 (1)老年人“养儿防老”的观念正在发生转变,且这一养老观念的转变与老年人的性别和文化程度无关.(2)养老观念的变化使老年人的幸福感指向的对象也发生了变化,不再认为一定要与子女住在一起安度晚年才能幸福.由此导致老年人对养老居住方式有了不同的选择.A study on the relationship between adaptive ability and home environment in middle school
Li, G., & Xu, V. S. (n.d.).Publication year
1999Journal title
In Learning and ResearchPage(s)
45