Yajun Mei
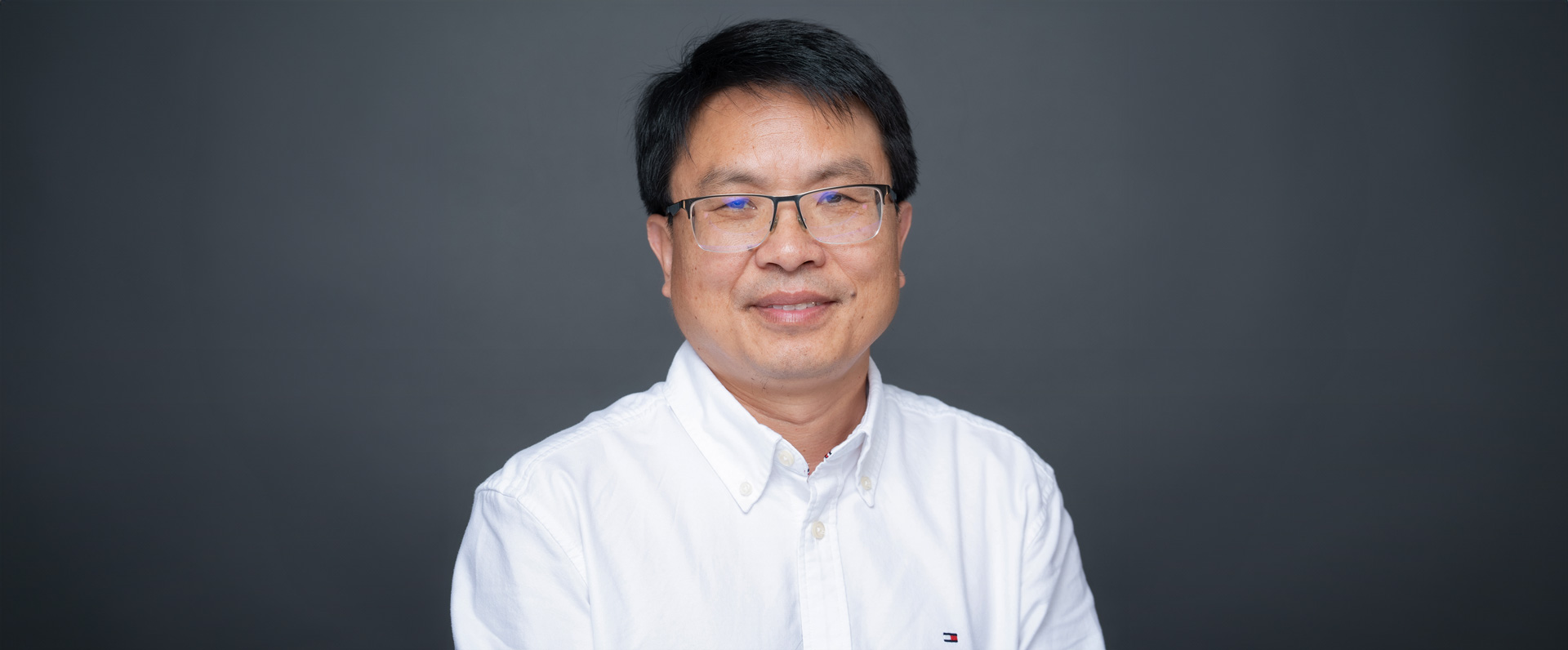
Professor of Biostatistics
-
Professional overview
-
Yajun Mei is a Professor of Biostatistics at NYU/GPH, starting from July 1, 2024. He received the B.S. degree in Mathematics from Peking University, Beijing, China, in 1996, and the Ph.D. degree in Mathematics with a minor in Electrical Engineering from the California Institute of Technology, Pasadena, CA, USA, in 2003. He was a Postdoc in Biostatistics in the renowned Fred Hutch Cancer Center in Seattle, WA during 2003 and 2005. Prior to joining NYU, Dr. Mei was an Assistant/Associate/Full Professor in H. Milton Stewart School of Industrial and Systems Engineering at the Georgia Institute of Technology, Atlanta, GA for 18 years from 2006 to 2024, and had been a co-director of Biostatistics, Epidemiology, and Study Design (BERD) of Georgia CTSA since 2018.
Dr. Mei’s research interests are statistics, machine learning, and data science, and their applications in biomedical science and public health, particularly, streaming data analysis, sequential decision/design, change-point problems, precision/personalized medicine, hot-spots detection for infectious diseases, longitudinal data analysis, bioinformatics, and clinical trials. His work has received several recognitions including Abraham Wald Prizes in Sequential Analysis in both 2009 and 2024, NSF CAREER Award in 2010, an elected Fellow of American Statistical Association (ASA) in 2023, and multiple best paper awards.
-
Education
-
BS, Mathematics, Peking UniversityPhD, Mathematics, California Institute of Technology
-
Honors and awards
-
Fellow of American Statistical Association (2023)Star Research Achievement Award, 2021 Virtual Critical Care Congress (2021)Best Paper Competition Award, Quality, Statistics & Reliability of INFORMS (2020)Bronze Snapshot Award, Society of Critical Care Medicine (2019)NSF Career AwardThank a Teacher Certificate, Center for Teaching and Learning (2011201220162020202120222023)Abraham Wald Prize (2009)Best Paper Award, 11th International Conference on Information Fusion (2008)New Researcher Fellow, Statistical and Applied Mathematical Sciences Institute (2005)Fred Hutchinson SPAC Travel Award to attend 2005 Joint Statistical Meetings, Minneapolis, MN (2005)Travel Award to 8th New Researchers Conference, Minneapolis, MN (2005)Travel Award to IEEE International Symposium on Information Theory, Chicago, IL (2004)Travel Award to IPAM workshop on inverse problem, UCLA, Los Angeles, CA (2003)Fred Hutchinson SPAC Course Scholarship (2003)Travel Award to the SAMSI workshop on inverse problem, Research Triangular Park, NC (2002)
-
Publications
Publications
Editorial: Mathematical Fundamentals of Machine Learning
Glickenstein, D., Hamm, K., Huo, X., Mei, Y., & Stoll, M. (n.d.).Publication year
2021Journal title
Frontiers in Applied Mathematics and StatisticsVolume
7Nonparametric monitoring of multivariate data via KNN learning
Li, W., Zhang, C., Tsung, F., & Mei, Y. (n.d.).Publication year
2021Journal title
International Journal of Production ResearchVolume
59Issue
20Page(s)
6311-6326AbstractProcess monitoring of multivariate quality attributes is important in many industrial applications, in which rich historical data are often available thanks to modern sensing technologies. While multivariate statistical process control (SPC) has been receiving increasing attention, existing methods are often inadequate as they are sensitive to the parametric model assumptions of multivariate data. In this paper, we propose a novel, nonparametric k-nearest neighbours empirical cumulative sum (KNN-ECUSUM) control chart that is a machine-learning-based black-box control chart for monitoring multivariate data by utilising extensive historical data under both in-control and out-of-control scenarios. Our proposed method utilises the k-nearest neighbours (KNN) algorithm for dimension reduction to transform multivariate data into univariate data and then applies the CUSUM procedure to monitor the change on the empirical distribution of the transformed univariate data. Extensive simulation studies and a real industrial example based on a disk monitoring system demonstrate the robustness and effectiveness of our proposed method.Optimum Multi-Stream Sequential Change-Point Detection with Sampling Control
Xu, Q., Mei, Y., & Moustakides, G. V. (n.d.).Publication year
2021Journal title
IEEE Transactions on Information TheoryVolume
67Issue
11Page(s)
7627-7636AbstractIn multi-stream sequential change-point detection it is assumed that there are M processes in a system and at some unknown time, an occurring event changes the distribution of the samples of a particular process. In this article, we consider this problem under a sampling control constraint when one is allowed, at each point in time, to sample a single process. The objective is to raise an alarm as quickly as possible subject to a proper false alarm constraint. We show that under sampling control, a simple myopic-sampling-based sequential change-point detection strategy is second-order asymptotically optimal when the number M of processes is fixed. This means that the proposed detector, even by sampling with a rate 1/M of the full rate, enjoys the same detection delay, up to some additive finite constant, as the optimal procedure. Simulation experiments corroborate our theoretical results.Quantitation of lymphatic transport mechanism and barrier influences on lymph node-resident leukocyte access to lymph-borne macromolecules and drug delivery systems
Archer, P. A., Sestito, L. F., Manspeaker, M. P., O’Melia, M. J., Rohner, N. A., Schudel, A., Mei, Y., & Thomas, S. N. (n.d.).Publication year
2021Journal title
Drug Delivery and Translational ResearchVolume
11Issue
6Page(s)
2328-2343AbstractLymph nodes (LNs) are tissues of the immune system that house leukocytes, making them targets of interest for a variety of therapeutic immunomodulation applications. However, achieving accumulation of a therapeutic in the LN does not guarantee equal access to all leukocyte subsets. LNs are structured to enable sampling of lymph draining from peripheral tissues in a highly spatiotemporally regulated fashion in order to facilitate optimal adaptive immune responses. This structure results in restricted nanoscale drug delivery carrier access to specific leukocyte targets within the LN parenchyma. Herein, a framework is presented to assess the manner in which lymph-derived macromolecules and particles are sampled in the LN to reveal new insights into how therapeutic strategies or drug delivery systems may be designed to improve access to dLN-resident leukocytes. This summary analysis of previous reports from our group assesses model nanoscale fluorescent tracer association with various leukocyte populations across relevant time periods post administration, studies the effects of bioactive molecule NO on access of lymph-borne solutes to dLN leukocytes, and illustrates the benefits to leukocyte access afforded by lymphatic-targeted multistage drug delivery systems. Results reveal trends consistent with the consensus view of how lymph is sampled by LN leukocytes resulting from tissue structural barriers that regulate inter-LN transport and demonstrate how novel, engineered delivery systems may be designed to overcome these barriers to unlock the therapeutic potential of LN-resident cells as drug delivery targets.Routine Use of Contrast on Admission Transthoracic Echocardiography for Heart Failure Reduces the Rate of Repeat Echocardiography during Index Admission
Lee, K. C., Liu, S., Callahan, P., Green, T., Jarrett, T., Cochran, J. D., Mei, Y., Mobasseri, S., Sayegh, H., Rangarajan, V., Flueckiger, P., & Vannan, M. A. (n.d.).Publication year
2021Journal title
Journal of the American Society of EchocardiographyVolume
34Issue
12Page(s)
1253-1261.e4AbstractBackground: The authors retrospectively evaluated the impact of ultrasound enhancing agent (UEA) use in the first transthoracic echocardiographic (TTE) examination, regardless of baseline image quality, on the number of repeat TTEs and length of stay (LOS) during a heart failure (HF) admission. Methods: There were 9,115 HF admissions associated with admission TTE examinations over a 4-year period (5,337 men; mean age, 67.6 ± 15.0 years). Patients were grouped into those who received UEAs (contrast group) in the first TTE study and those who did not (noncontrast group). Repeat TTE examinations were classified as justified if performed for concrete clinical indications during hospitalization. Results: In the 9,115 admissions for HF (5,600 in the contrast group, 3,515 in the noncontrast group), 927 patients underwent repeat TTE studies (505 in the contrast group, 422 in the noncontrast group), which were considered justified in 823 patients. Of the 104 patients who underwent unjustified repeat TTE studies, 80 (76.7%) belonged to the noncontrast group and 24 to the contrast group. Also, UEA use increased from 50.4% in 2014 to 74.3%, and the rate of unjustified repeat studies decreased from 1.3% to 0.9%. The rates of unjustified repeat TTE imaging were 2.3% and 0.4% (in the noncontrast and contrast groups, respectively), and patients in the contrast group were less likely to undergo unjustified repeat examinations (odds ratio, 0.18; 95% CI, 0.12–0.29; P <.0001). The mean LOS was significantly lower in the contrast group (9.5 ± 10.5 vs 11.1 ± 13.7 days). The use of UEA in the first TTE study was also associated with reduced LOS (linear regression, β1 = −0.47, P =.036), with 20% lower odds for odds of prolonged (>6 days) LOS. Conclusions: The routine use of UEA in the first TTE examination for HF irrespective of image quality is associated with reduced unjustified repeat TTE testing and may reduce LOS during an index HF admission.Single and multiple change-point detection with differential privacy
Zhang, W., Krehbiel, S., Tuo, R., Mei, Y., & Cummings, R. (n.d.).Publication year
2021Journal title
Journal of Machine Learning ResearchVolume
22AbstractThe change-point detection problem seeks to identify distributional changes at an unknown change-point k* in a stream of data. This problem appears in many important practical settings involving personal data, including biosurveillance, fault detection, finance, signal detection, and security systems. The field of differential privacy offers data analysis tools that provide powerful worst-case privacy guarantees. We study the statistical problem of change-point detection through the lens of differential privacy. We give private algorithms for both online and offine change-point detection, analyze these algorithms theoretically, and provide empirical validation of our results.Glucose Variability as Measured by Inter-measurement Percentage Change is Predictive of In-patient Mortality in Aneurysmal Subarachnoid Hemorrhage
Sadan, O., Feng, C., Vidakovic, B., Mei, Y., Martin, K., Samuels, O., & Hall, C. L. (n.d.).Publication year
2020Journal title
Neurocritical CareVolume
33Issue
2Page(s)
458-467AbstractBackground: Critically ill aneurysmal subarachnoid hemorrhage (aSAH) patients suffer from systemic complications at a high rate. Hyperglycemia is a common intensive care unit (ICU) complication and has become a focus after aggressive glucose management was associated with improved ICU outcomes. Subsequent research has suggested that glucose variability, not a specific blood glucose range, may be a more appropriate clinical target. Glucose variability is highly correlated to poor outcomes in a wide spectrum of critically ill patients. Here, we investigate the changes between subsequent glucose values termed “inter-measurement difference,” as an indicator of glucose variability and its association with outcomes in patients with aSAH. Methods: All SAH admissions to a single, tertiary referral center between 2002 and 2016 were screened. All aneurysmal cases who had more than 2 glucose measurements were included (n = 2451). We calculated several measures of variability, including simple variance, the average consecutive absolute change, average absolute change by time difference, within subject variance, median absolute deviation, and average or median consecutive absolute percentage change. Predictor variables also included admission Hunt and Hess grade, age, gender, cardiovascular risk factors, and surgical treatment. In-patient mortality was the main outcome measure. Results: In a multiple regression analysis, nearly all forms of glucose variability calculations were found to be correlated with in-patient mortality. The consecutive absolute percentage change, however, was most predictive: OR 5.2 [1.4–19.8, CI 95%] for percentage change and 8.8 [1.8–43.6] for median change, when controlling for the defined predictors. Survival to ICU discharge was associated with lower glucose variability (consecutive absolute percentage change 17% ± 9%) compared with the group that did not survive to discharge (20% ± 15%, p ' 0.01). Interestingly, this finding was not significant in patients with pre-admission poorly controlled diabetes as indicated by HbA1c (OR 0.45 [0.04–7.18], by percentage change). The effect is driven mostly by non-diabetic patients or those with well-controlled diabetes. Conclusions: Reduced glucose variability is highly correlated with in-patient survival and long-term mortality in aSAH patients. This finding was observed in the non-diabetic and well-controlled diabetic patients, suggesting a possible benefit for personalized glucose targets based on baseline HbA1c and minimizing variability. The inter-measure percentage change as an indicator of glucose variability is not only predictive of outcome, but is an easy-to-use tool that could be implemented in future clinical trials.Improved performance properties of the CISPRT algorithm for distributed sequential detection
Liu, K., & Mei, Y. (n.d.).Publication year
2020Journal title
Signal ProcessingVolume
172AbstractIn distributed sequential detection problems, local sensors observe raw local observations over time, and are allowed to communicate local information with their immediate neighborhood at each time step so that the sensors can work together to make a quick but accurate decision when testing binary hypotheses on the true raw sensor distributions. One interesting algorithm is the Consensus-Innovation Sequential Probability Ratio Test (CISPRT) algorithm proposed by Sahu and Kar (IEEE Trans. Signal Process., 2016). In this article, we present improved finite-sample properties on error probabilities and expected sample sizes of the CISPRT algorithm for Gaussian data in term of network connectivity, and more importantly, derive its sharp first-order asymptotic properties in the classical asymptotic regime when Type I and II error probabilities go to 0. The usefulness of our theoretical results are validated through numerical simulations.Wavelet-Based Robust Estimation of Hurst Exponent with Application in Visual Impairment Classification
Feng, C., Mei, Y., & Vidakovic, B. (n.d.).Publication year
2020Journal title
Journal of Data ScienceVolume
18Issue
4Page(s)
581-605AbstractPupillary response behavior (PRB) refers to changes in pupil diameter in response to simple or complex stimuli. There are underlying, unique patterns hidden within complex, high-frequency PRB data that can be utilized to classify visual impairment, but those patterns cannot be described by traditional summary statistics. For those complex high-frequency data, Hurst exponent, as a measure of long-term memory of time series, becomes a powerful tool to detect the muted or irregular change patterns. In this paper, we proposed robust estimators of Hurst exponent based on non-decimated wavelet transforms. The properties of the proposed estimators were studied both theoretically and numerically. We applied our methods to PRB data to extract the Hurst exponent and then used it as a predictor to classify individuals with different degrees of visual impairment. Compared with other standard wavelet-based methods, our methods reduce the variance of the estimators and increase the classification accuracy.Optimal Stopping for Interval Estimation in Bernoulli Trials
Yaacoub, T., Moustakides, G. V., & Mei, Y. (n.d.).Publication year
2019Journal title
IEEE Transactions on Information TheoryVolume
65Issue
5Page(s)
3022-3033AbstractWe propose an optimal sequential methodology for obtaining confidence intervals for a binomial proportion \theta. Assuming that an independent and identically distributed sequence of Bernoulli ( \theta ) trials is observed sequentially, we are interested in designing: 1) a stopping time T that will decide the best time to stop sampling the process and 2) an optimum estimator \hat{{\theta}}-{{T}} that will provide the optimum center of the interval estimate of \theta. We follow a semi-Bayesian approach, where we assume that there exists a prior distribution for \theta , and our goal is to minimize the average number of samples while we guarantee a minimal specified coverage probability level. The solution is obtained by applying standard optimal stopping theory and computing the optimum pair (T,\hat{{\theta }}-{{T}}) numerically. Regarding the optimum stopping time component T , we demonstrate that it enjoys certain very interesting characteristics not commonly encountered in solutions of other classical optimal stopping problems. In particular, we prove that, for a particular prior (beta density), the optimum stopping time is always bounded from above and below; it needs to first accumulate a sufficient amount of information before deciding whether or not to stop, and it will always terminate before some finite deterministic time. We also conjecture that these properties are present with any prior. Finally, we compare our method with the optimum fixed-sample-size procedure as well as with existing alternative sequential schemes.Scalable sum-shrinkage schemes for distributed monitoring large-scale data streams
Liu, K., Zhang, R., & Mei, Y. (n.d.).Publication year
2019Journal title
Statistica SinicaVolume
29Issue
1Page(s)
1-22AbstractIn this article, we investigate the problem of monitoring independent large-scale data streams where an undesired event may occur at some unknown time and affect only a few unknown data streams. Motivated by parallel and distributed computing, we propose to develop scalable global monitoring schemes by parallel running local detection procedures and by using the sum of the shrinkage transformation of local detection statistics as a global statistic to make a decision. The usefulness of our proposed SUM-Shrinkage approach is illustrated in an example of monitoring large-scale independent normally distributed data streams when the local post-change mean shifts are unknown and can be positive or negative.Tandem-width sequential confidence intervals for a Bernoulli proportion
Yaacoub, T., Goldsman, D., Mei, Y., & Moustakides, G. V. (n.d.).Publication year
2019Journal title
Sequential AnalysisVolume
38Issue
2Page(s)
163-183AbstractWe propose a two-stage sequential method for obtaining tandem-width confidence intervals for a Bernoulli proportion p. The term “tandem-width” refers to the fact that the half-width of the 100(1 - α)% confidence interval is not fixed beforehand; it is instead required to satisfy two different half-width upper bounds, h0 and h1, depending on the (unknown) values of p. To tackle this problem, we first propose a simple but useful sequential method for obtaining fixed-width confidence intervals for p, whose stopping rule is based on the minimax estimator of p. We observe Bernoulli(p) trials sequentially, and for some fixed half-width h = h0 or h1, we develop a stopping time T such that the resulting confidence interval for p, [(Formula presented.)], covers the parameter with confidence at least 100(1 - α)% where (Formula presented.) is the maximum likelihood estimator of p at time T. Furthermore, we derive theoretical properties of our proposed fixed-width and tandem-width methods and compare their performances with existing alternative sequential schemes. The proposed minimax-based fixed-width method performs similarly to alternative fixed-width methods, while being easier to implement in practice. In addition, the proposed tandem-width method produces effective savings in sample size compared to the fixed-width counterpart and provides excellent results for scientists to use when no prior knowledge of p is available.Asymptotic statistical properties of communication-efficient quickest detection schemes in sensor networks
Zhang, R., & Mei, Y. (n.d.).Publication year
2018Journal title
Sequential AnalysisVolume
37Issue
3Page(s)
375-396AbstractThe quickest change detection problem is studied in a general context of monitoring a large number K of data streams in sensor networks when the “trigger event” may affect different sensors differently. In particular, the occurring event might affect some unknown, but not necessarily all, sensors and also could have an immediate or delayed impact on those affected sensors. Motivated by censoring sensor networks, we develop scalable communication-efficient schemes based on the sum of those local cumulative sum (CUSUM) statistics that are “large” under either hard, soft, or order thresholding rules. Moreover, we provide the detection delay analysis of these communication-efficient schemes in the context of monitoring K independent data streams and establish their asymptotic statistical properties under two regimes: one is the classical asymptotic regime when the dimension K is fixed, and the other is the modern asymptotic regime when the dimension K goes to ∞ Our theoretical results illustrate the deep connections between communication efficiency and statistical efficiency.Thresholded Multivariate Principal Component Analysis for Phase I Multichannel Profile Monitoring
Wang, Y., Mei, Y., & Paynabar, K. (n.d.).Publication year
2018Journal title
TechnometricsVolume
60Issue
3Page(s)
360-372AbstractMonitoring multichannel profiles has important applications in manufacturing systems improvement, but it is nontrivial to develop efficient statistical methods because profiles are high-dimensional functional data with intrinsic inner- and interchannel correlations, and that the change might only affect a few unknown features of multichannel profiles. To tackle these challenges, we propose a novel thresholded multivariate principal component analysis (PCA) method for multichannel profile monitoring. Our proposed method consists of two steps of dimension reduction: It first applies the functional PCA to extract a reasonably large number of features under the in-control state, and then uses the soft-thresholding techniques to further select significant features capturing profile information under the out-of-control state. The choice of tuning parameter for soft-thresholding is provided based on asymptotic analysis, and extensive numerical studies are conducted to illustrate the efficacy of our proposed thresholded PCA methodology.Precision in the specification of ordinary differential equations and parameter estimation in modeling biological processes
Holte, S. E., & Mei, Y. (n.d.). In Quantitative Methods for HIV/AIDS Research (1–).Publication year
2017Page(s)
257-281AbstractIn recent years, the use of differential equations to describe the dynamics of within-host viral infections, most frequently HIV-1 or Hepatitis B or C dynamics, has become quite common. The pioneering work described in [1,2,3,4] provided estimates of both the HIV-1 viral clearance rate, c, and infected cell turnover rate, δ, and revealed that while it often takes years for HIV-1 infection to progress to AIDS, the virus is replicating rapidly and continuously throughout these years of apparent latent infection. In addition, at least two compartments of viral-producing cells that decay at different rates were identified. Estimates of infected cell decay and viral clearance rates dramatically changed the understanding of HIV replication, etiology, and pathogenesis. Since that time, models of this type have been used extensively to describe and predict both in vivo viral and/or immune system dynamics and the transmission of HIV throughout a population. However, there are both mathematical and statistical challenges associated with models of this type, and the goal of this chapter is to describe some of these as well as offer possible solutions or options. In particular statistical aspects associated with parameter estimation, model comparison and study design will be described. Although the models developed by Perelson et al. [3,4] are relatively simple and were developed nearly 20 years ago, these models will be used in this chapter to demonstrate concepts in a relatively simple setting. In the first section, a statistical approach for model comparison is described using the model developed in [4] as the null hypothesis model for formal statistical comparison to an alternative model. In the next section, the concept of the mathematical sensitivity matrix and its relationship to the Fisher information matrix (FIM) will be described, and will be used to demonstrate how to evaluate parameter identifiability in ordinary differential equation (ODE) models. The next section demonstrates how to determine what types of additional data are required to address the problem of nonidentifiable parameters in ODE models. Examples are provided to demonstrate these concepts. The chapter ends with some recommendations.Search for evergreens in science: A functional data analysis
Zhang, R., Wang, J., & Mei, Y. (n.d.).Publication year
2017Journal title
Journal of InformetricsVolume
11Issue
3Page(s)
629-644AbstractEvergreens in science are papers that display a continual rise in annual citations without decline, at least within a sufficiently long time period. Aiming to better understand evergreens in particular and patterns of citation trajectory in general, this paper develops a functional data analysis method to cluster citation trajectories of a sample of 1699 research papers published in 1980 in the American Physical Society (APS) journals. We propose a functional Poisson regression model for individual papers’ citation trajectories, and fit the model to the observed 30-year citations of individual papers by functional principal component analysis and maximum likelihood estimation. Based on the estimated paper-specific coefficients, we apply the K-means clustering algorithm to cluster papers into different groups, for uncovering general types of citation trajectories. The result demonstrates the existence of an evergreen cluster of papers that do not exhibit any decline in annual citations over 30 years.Discussion on “Sequential detection/isolation of abrupt changes” by Igor V. Nikiforov
Liu, K., & Mei, Y. (n.d.).Publication year
2016Journal title
Sequential AnalysisVolume
35Issue
3Page(s)
316-319AbstractIn this interesting article, Professor Nikiforov reviewed the current state of quickest change detection/isolation problem. In our discussion of his article we focus on the concerns and the opportunities of the subfield of quickest change detection or, more generally, sequential methodologies, in the modern information age.Effect of bivariate data's correlation on sequential tests of circular error probability
Li, Y., & Mei, Y. (n.d.).Publication year
2016Journal title
Journal of Statistical Planning and InferenceVolume
171Page(s)
99-114AbstractThe problem of evaluating a military or GPS/GSM system's precision quality is considered in this article, where one sequentially observes bivariate normal data (Xi, Yi)'s and wants to test hypotheses on the circular error probability (CEP) or the probability of nonconforming, i.e., the probabilities of the system hitting or missing a pre-specified disk target. In such a problem, we first consider a sequential probability ratio test (SPRT) developed under the erroneous assumption of the correlation coefficient ρ=0, and investigate its properties when the true ρ≠0. It was shown that at least one of the Type I and Type II error probabilities would be larger than the required ones if the true ρ≠0, and for the detailed effects, exp-2≈0.1353 turns out to be a critical value for the hypothesized probability of nonconforming. Moreover, we propose several sequential tests when the correlation coefficient ρ is unknown, and among these tests, the method of generalized sequential likelihood ratio test (GSLRT) in Bangdiwala (1982) seems to work well.Symmetric directional false discovery rate control
Holte, S. E., Lee, E. K., & Mei, Y. (n.d.).Publication year
2016Journal title
Statistical MethodologyVolume
33Page(s)
71-82AbstractThis research is motivated from the analysis of a real gene expression data that aims to identify a subset of “interesting” or “significant” genes for further studies. When we blindly applied the standard false discovery rate (FDR) methods, our biology collaborators were suspicious or confused, as the selected list of significant genes was highly unbalanced: there were ten times more under-expressed genes than the over-expressed genes. Their concerns led us to realize that the observed two-sample t-statistics were highly skewed and asymmetric, and thus the standard FDR methods might be inappropriate. To tackle this case, we propose a symmetric directional FDR control method that categorizes the genes into “over-expressed” and “under-expressed” genes, pairs “over-expressed” and “under-expressed” genes, defines the p-values for gene pairs via column permutations, and then applies the standard FDR method to select “significant” gene pairs instead of “significant” individual genes. We compare our proposed symmetric directional FDR method with the standard FDR method by applying them to simulated data and several well-known real data sets.An Adaptive Sampling Strategy for Online High-Dimensional Process Monitoring
Liu, K., Mei, Y., & Shi, J. (n.d.).Publication year
2015Journal title
TechnometricsVolume
57Issue
3Page(s)
305-319AbstractTemporally and spatially dense data-rich environments provide unprecedented opportunities and challenges for effective process control. In this article, we propose a systematic and scalable adaptive sampling strategy for online high-dimensional process monitoring in the context of limited resources with only partial information available at each acquisition time. The proposed adaptive sampling strategy includes a broad range of applications: (1) when only a limited number of sensors is available; (2) when only a limited number of sensors can be in "ON" state in a fully deployed sensor network; and (3) when only partial data streams can be analyzed at the fusion center due to limited transmission and processing capabilities even though the full data streams have been acquired remotely. A monitoring scheme of using the sum of top-r local CUSUM statistics is developed and named as "TRAS" (top-r based adaptive sampling), which is scalable and robust in detecting a wide range of possible mean shifts in all directions, when each data stream follows a univariate normal distribution. Two properties of this proposed method are also investigated. Case studies are performed on a hot-forming process and a real solar flare process to illustrate and evaluate the performance of the proposed method.Large-Scale Multi-Stream Quickest Change Detection via Shrinkage Post-Change Estimation
Wang, Y., & Mei, Y. (n.d.).Publication year
2015Journal title
IEEE Transactions on Information TheoryVolume
61Issue
12Page(s)
6926-6938AbstractThe quickest change detection problem is considered in the context of monitoring large-scale independent normal distributed data streams with possible changes in some of the means. It is assumed that for each individual local data stream, either there are no local changes, or there is a big local change that is larger than a pre-specified lower bound. Two different types of scenarios are studied: one is the sparse post-change case when the unknown number of affected data streams is much smaller than the total number of data streams, and the other is when all local data streams are affected simultaneously although not necessarily identically. We propose a systematic approach to develop efficient global monitoring schemes for quickest change detection by combining hard thresholding with linear shrinkage estimators to estimating all post-change parameters simultaneously. Our theoretical analysis demonstrates that the shrinkage estimation can balance the tradeoff between the first-order and second-order terms of the asymptotic expression on the detection delays, and our numerical simulation studies illustrate the usefulness of shrinkage estimation and the challenge of Monte Carlo simulation of the average run length to false alarm in the context of online monitoring large-scale data streams.Quickest Change Detection and Kullback-Leibler Divergence for Two-State Hidden Markov Models
Fuh, C. D., & Mei, Y. (n.d.).Publication year
2015Journal title
IEEE Transactions on Signal ProcessingVolume
63Issue
18Page(s)
4866-4878AbstractIn this paper, the quickest change detection problem is studied in two-state hidden Markov models (HMM), where the vector parameter θ of the HMM changes from θ0 to θ1 at some unknown time, and one wants to detect the true change as quickly as possible while controlling the false alarm rate. It turns out that the generalized likelihood ratio (GLR) scheme, while theoretically straightforward, is generally computationally infeasible for the HMM. To develop efficient but computationally simple schemes for the HMM, we first discuss a subtlety in the recursive form of the generalized likelihood ratio (GLR) scheme for the HMM. Then we show that the recursive CUSUM scheme proposed in Fuh (Ann. Statist., 2003) can be regarded as a quasi-GLR scheme for pseudo post-change hypotheses with certain dependence structure between pre- and postchange observations. Next, we extend the quasi-GLR idea to propose recursive score schemes in the scenario when the postchange parameter θ1 of the HMM involves a real-valued nuisance parameter. Finally, the Kullback-Leibler (KL) divergence plays an essential role in the quickest change detection problem and many other fields, however it is rather challenging to numerically compute it in HMMs. Here we develop a non-Monte Carlo method that computes the KL divergence of two-state HMMs via the underlying invariant probability measure, which is characterized by the Fredholm integral equation. Numerical study demonstrates an unusual property of the KL divergence for HMM that implies the severe effects of misspecifying the postchange parameter for the HMM.Discussion on "Change-Points: From Sequential Detection to Biology and Back" by David O. Siegmund
Mei, Y. (n.d.).Publication year
2013Journal title
Sequential AnalysisVolume
32Issue
1Page(s)
32-35AbstractIn his interesting paper, Professor Siegmund illustrates that the problem formulations and methodologies are generally transferable between off-line and on-line settings of change-point problems. In our discussion of his paper, we echo his thoughts with our own experiences.Quantization effect on the log-likelihood ratio and its application to decentralized sequential detection
Wang, Y., & Mei, Y. (n.d.).Publication year
2013Journal title
IEEE Transactions on Signal ProcessingVolume
61Issue
6Page(s)
1536-1543AbstractIt is well known that quantization cannot increase the Kullback-Leibler divergence which can be thought of as the expected value or first moment of the log-likelihood ratio. In this paper, we investigate the quantization effects on the second moment of the log-likelihood ratio. It is shown via the convex domination technique that quantization may result in an increase in the case of the second moment, but the increase is bounded above by 2/e. The result is then applied to decentralized sequential detection problems not only to provide simpler sufficient conditions for asymptotic optimality theories in the simplest models, but also to shed new light on more complicated models. In addition, some brief remarks on other higher-order moments of the log-likelihood ratio are also provided.A multistage procedure for decentralized sequential multi-hypothesis testing problems
Wang, Y., & Mei, Y. (n.d.).Publication year
2012Journal title
Sequential AnalysisVolume
31Issue
4Page(s)
505-527AbstractWe studied the problem of sequentially testing M ≥ 2 hypotheses with a decentralized sensor network system. In such a system, the local sensors observe raw data and then send quantized observations to a fusion center, which makes a final decision regarding hypothesis is true. Motivated by the two-stage tests in Wang and Mei (2011), we propose a multistage decentralized sequential test that provides multiple opportunities for the local sensors to adjust to the optimal local quantizers. It is demonstrated that when the hypothesis testing problem is asymmetric, the multistage test is second-order asymptotically optimal. Even though this result constitutes an interesting theoretical improvement over twostage tests that can enjoy only first-order asymptotic optimality, the corresponding practical merits seem to be only marginal. Indeed, performance gains over two-stage procedures with carefully selected thresholds are small.